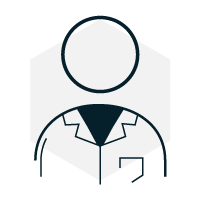
Author: Ritam Bhandari
Hult International Business School
INTRODUCTION
Let us first understand what is circular economy. The circular economy is a system in which materials are never wasted and nature is replenished. A circular economy keeps items and materials in circulation through activities such as maintenance, reuse, refurbishing, remanufacturing, recycling, and composting. The circular economy addresses climate change and other global issues such as biodiversity loss, waste, and pollution by separating economic activity from the consumption of scarce resources. (Macarthur et al., 2022) Humans’ perspective of resource management and economic growth has drastically evolved as a result of the circular economy. The linkage between circular economy and sustainability needs to be well understood. The circular economy is a way to achieve SDGs. Sustainability is a larger umbrella which has a circular economy as its handle. As per research, it is shown that CE is the tool that needs to be used to achieve sustainable development, especially in terms of the environment(M. Walker ., 2018) which we are going to focus on in this research. With the start of the Industrial Revolution, the take-make-dispose paradigm of resource consumption has come to represent the linear economy. However, with the introduction of mass manufacturing, consumption, and trade globalisation, it quickly began to drastically decline, giving rise to the Circular economy (CE). The term “circular economy” (CE) was initially used by Pearce and Turner (1990) in their work “Economics of Natural Resources and Environment.” They claimed that the current economic system should be changed to a circular economy because producing activities are constantly generating waste and pollution, endangering the environment so the system should be transformed into a circular one by considering waste as a source for more resources. (Andrade et al., 2022))
There are still several gaps and challenges impeding the digital technology-aided circular business model. There are still a lot of problems related to information that act as barriers to the integration of technology and the circular economy. Among these include inadequate information, high transaction and search expenses and a lack of knowledge. Data integration issues are also overlooked while considering the role of digital solutions in the circular economy. This is an essential issue as it is not always clear how to utilize data to promote the shift from a linear economy to a more circular one. Data integration is important for businesses as it combines the information sources with the business goals of the stakeholders involved.
Expanding on the issues mentioned above, the lack of information and data about various products and supply chains can cause problems as without relevant data it is difficult to manage the resources properly. The existing knowledge gap can also prevent businesses from reducing their waste generation. The costs required to obtain and utilize relevant data can be very high, especially for smaller companies and start-ups. This not only includes the money associated but also the time and energy required to evaluate the data. The integration of data is also an essential issue as it is not always clear how to utilize data to promote the shift from a linear economy to a more circular one. Various stakeholders use different formats, standards, tools and platforms for data integration causing difficulties in decision making.
AIM:
The topic of research the author proposes is a study to investigate the impact of data science in better adoption and implementation of circular economy in manufacturing. The study is going to look into the different variables and their extent of impact on the adoption of circular economy in manufacturing.
Research question –
What is the influence of data science on circular economy in manufacturing?
Objectives:
- To gain insights and explore the scope of the circular economy.
- To identify and investigate how data science impacts the circular economy.
- To establish a relationship between data science and circular economy in manufacturing
- To observe the influence of data science in circular economy in manufacturing
2. LITERATURE REVIEW
2.1. Circular economy
The circular economy is a model which is framed to keep existing resources in use and increase their lifespan for as long as possible. Unlike traditional linear economy which follows a “take-make-dispose” model where products are disposed of after being used, circular economy aims at keeping products and materials in use and extracting maximum value from them by eliminating the waste produced by them. Furthermore, the circular economy revolves around product sustainability design development, reuse, remanufacture, and recycling principles. The process of circular economy starts by reducing unnecessary consumption and product design development. Secondly, efforts are made to increase the product lifecycle and reduce the need for new raw materials by refurbishment, remanufacturing, and repurposing. In the end, materials which can be recycled are taken out from products whose lifecycle has ended, and the emphasis on new virgin materials required as raw material is reduced. Product sustainability design refers to the creation of new products in such a way that their negative effects on the environment can be minimized by following various steps such as material selection, and energy efficiency. Remanufacturing is the process of restoring old and used products to new-like conditions so that the need for new materials and extra energy can be reduced.
2.2 Development of circular economy over a period
(1990s): Attempts were being made to frame the concept of “Circular economy” by one of the well-known economists, D. Pierce who was later joined by K. Turner for the same. Other terms like “cradle-to-cradle” and industrial ecology were also put forward, which were identical and awareness regarding the change of approach to using materials started developing. “Eco-industrial parks were developed in North and South America, Southeast Asia, Europe and southern Africa during the 1990s”. Talking about the example of Copenhagen, the waste of coal in one of the power plants started being used as raw materials in fertilizers.
(2000 to 2010): A need to change production and consumption methods along with conserving natural resources was felt by some of the world’s greatest political leaders. Germany introduced its “Closed Substance Cycle Management Act” and Japan also added basic laws to approach a sustainable economy and recycle-based society in 2000. In addition to this, China became the world’s first country to adopt legislation on circular economy in 2002 and these changes were made in its 12th Five-Year Plan.
(2010 to present): The main reason for the shift from a linear model to a circular model of the economy is to ensure the sustainable development of the world in the context of the fourth industrial revolution. Moreover, it has been studied that this paradigm shift from and abandonment of “single-use” resources has the potential to generate economic benefits worth $4.5 trillion by 2030. Adding on, 44% of the Fortune Global top-100 companies have already adopted the mechanisms of circular economy. (Walter et al ., 2018) One of the major factors which led to consumers rethink their approach towards sustainability and widen the use of digital technology in the circular economy was COVID-19, significantly increasing consideration of the impact of their purchase on ESG (Environment, Social, and Corporate Governance). “The transition to a circular economy model in companies in these areas of economic activity will help reduce raw material costs, expand markets, improve brand reputation and dialogue with customers, increase their loyalty, create a competitive business model and much more.” According to a survey by e-commerce delivery platform Soundcloud, 38% of the respondents showed their willingness to pay an extra amount for the delivery of their goods in environmentally friendly vehicles.
2.3 Data Science and Technology
Now coming to digital technologies, IoT in business may help companies access real-time remote tracking of product location, status and consumption. IoT and other digital technologies help companies to gain knowledge about consumer behaviour and gain great opportunities thereby, changing the nature of the relationships between manufacturers and customers from negotiation to communication and increasing the scope of collaboration & partnership. As the products become smarter, manufacturers may upgrade their digital components such as firmware. Product sustainable upgradability will improve the transition to a Circular Economy by contrasting product obsolescence and its resulting material waste.
In written works, Big Data is usually defined through 4’s Volume, Variety, Velocity and Veracity. They are distinguished by i) huge volumes of data generated continuously, ii) different and unstructured forms, such as imaging, texting, etc. iii) high data generating frequency and iv) a good quality and a clear, validated application. As Big Data is massive and changes too quickly, it cannot be analysed using typical software or database procedures. In research, big data is usually seen as a legitimate strategy to facilitate better decision-making, when paired with the right analytical methods. To be more precise, analytics uses software and data mining techniques to create decision support systems and business intelligence to identify patterns in data and make decisions. Analyzing data may help in gaining the right implications and in terms of business it helps in gaining insights, for comprehensive decision-making. Thus, the combination of Big Data and analytics which leads in providing valuable insights leading to the right sustainable decision making may help management move closer to Circular Economy.
The adoption of a circular economy has given an advantage to various stakeholders across the businesses thus, promoting brand image, sustainability, economic and national growth. Shifting to circular business practices provides opportunities for cost optimization, and resource efficiency, and helps businesses escape price volatility. Ruling bodies and government benefit directly because of job creation and economic resilience features of the circular economy. Moreover, it allows them to reduce pollution levels and achieve their green initiatives and national sustainability goals much faster and more effectively as compared to linear manufacturing models. Consumers get the advantage of access to more durable, sustainable, and affordable products. Through processes such as remanufacturing and refurbishment, consumers can purchase the same products at much lower prices. Furthermore, consumers can track the supply chains of the products they are using because of transparent supply chain practices in a circular economy and make informed decisions regarding which products to purchase. For organizations, reduction in resource consumption, waste generation, and disposable costs leads to cost efficiency and cost optimization. Moreover, when processes like refurbishment and remanufacturing are implemented, the manufacturing costs of the business are also saved as the firm does not need to manufacture from scratch. Adding on, this also gives huge innovation opportunities to businesses as they can develop new products, and services by re-thinking about what can be further worked upon by using materials from other products. In addition to this, circular economy allows businesses for market differentiation as businesses which follow the model of circular economy, transparent supply chains, and fair sourcing of the materials can attract a loyal base of customers.
Although there are many advantages of circular economy as mentioned above, there are some challenges and limitations too that cannot be ignored. The standardization and interoperability of the models and formats of the data hinder the process of exchanging data seamlessly within multiple organizations. Moreover, organizations resist sharing their data due to extra efforts needed to transform the data and adopt other data modelling practices. This further leads to one bigger challenge, which is huge investments needed by organizations trying to adapt to the circular economy because of the upgradation and software cost needed for technology. Though AI (Artificial Intelligence), IOT (Internet of Things), and blockchain have emerged as promising technologies which help in the circular economy, the fact cannot be ignored that data privacy has also raised as a huge constraint because of huge concerns over privacy and security of data while using these technologies. Furthermore, strong intent of collaboration is the need of the hour due to the complex supply chain processes which include multiple stakeholders across the globe. Thus, product takeback, remanufacturing, and recycling which are the core of the circular economy become intensively complex and require transparency and collaboration.
Regulatory frameworks and policies also sometimes prove as a huge limitation in the implementation of digital technology in circular business models. The primary reasons can be complex regulatory requirements such as water and waste management or standardization of products, which can require huge resources to fulfil which can be troublesome for small and medium-sized enterprises (SMEs). Adding on, regulatory policies may lag to cope with fast-paced upcoming technologies like Artificial Intelligence and blockchain ultimately, creating a huge gap between the pace of both. Furthermore, many nations have outdated legal policies and regulations regarding the circular economy, which further creates an uncertain legal environment thus, preventing firms from investing and upgrading to circular economy models. There is a strong need for collaboration which enables circular-models organizations to assist governing bodies in framing and altering these policies. The digital skills gap from a global perspective is also a significant challenge as it reduces the effectiveness of technology-infused circular economy models. Human resources with expertise in various fields such as data analytics, supply chain optimization, data programming, and cybersecurity are most needed. But unfortunately, there is a shortage of these professionals in industries which are less-technology oriented such as manufacturing and agriculture. A potential solution can be collaboration between academics, training institutes and industry to bridge this gap.
2.4.Theories
Across the literature, the author has come across various theories which would help in establishing relationships between different variables within the theoretical framework. These theories are found to be aligned with the study being conducted.
2.4.1.Theory of decoupling
Disentangling “economic goods” from “environmental bads” has been suggested by the UN and the OECD as a way to achieve sustainability. The type of decoupling required for ecological sustainability is indicated by scientific consensus findings on resource consumption and environmental repercussions (such as greenhouse gas emissions): global, absolute, fast enough, and long enough. This objective provides a basis for classifying the various forms of decoupling according to their applicability. (UNEP,2011) The word refers to a situation in which economic progress occurs without a corresponding increase in the consumption of material resources or a detrimental influence on the environment, in contrast to most prior experiences (IRP, 2017, 23). The idea is particularly important for formulating policies that, in the first place, consider economic growth to be desirable or necessary, and, in the second place, acknowledge the unsustainable nature of the existing levels of material consumption and environmental harm brought on by the economy. Economic growth must be divorced from rising material consumption and environmental effects if it is to continue (OECD, 2001; UNEP, 2011). When one wishes to promote economic growth while acknowledging previous or present unsustainable development brought on by the economy, they can utilise the prospect of decoupling as support (Jackson and Victor, 2019; Hickel and Kallis, 2019)
2.4.2. Resource efficiency theory
Economic and environmental policy heavily weighs the importance of resource efficiency and the circular economy. The circular economy and resource efficiency are normative notions that advocate maximising prosperity and well-being while minimising losses and promoting enhanced material circulation while respecting the boundaries of the environment. Long-term advantages to society and the economy come from resource efficiency, which preserves the environment and lessens reliance on the natural resources that power the economy. In addition to assimilating air pollutants and wastes, ecosystems give society access to resources, food, clean water and air, as well as aesthetic and recreational enjoyment. Ecosystem collapse, deterioration, and depletion can be prevented with resource efficiency.
2.4.3. PSS theory
A product-service system is the outcome of an innovation strategy that moves the company’s emphasis from creating and marketing physical products to creating a system of products and services that work together to meet particular customer needs. A PSS is referred to as a Sustainable Product-Service System when it helps to reorient the present unsustainable trends in production and consumption practices. By using this strategy, a business offers extra services to ensure the product’s longevity and usefulness (also known as product life extension) before it is sold to a customer or consumer. Over a predetermined length of time, maintenance, repair, upgrading, and replacement services would all be included in a normal service contract. Following the conclusion of the contract, the PSS provider may take back the product, deciding about its possible sale or disposal.
2.4.4. Closed loop development theory
Closed-loop manufacturing systems work to achieve sustainability by concurrently advancing environmental and economic objectives. We point out that businesses can prevent a lot of harmful environmental effects, like waste, energy use, transportation, and packaging, by implementing closed-loop production methods. We present the idea of constructing closed-loop production systems using the framework of sustainable supply chain networks. The closing of process linkages across the businesses in a supply chain is made possible by the implementation of SSCN, which speeds up the shift from a flow economy to a circular economy. Circular economies are more sustainable, which eventually boosts both the economy and the environment. Designing is more than just picking and mixing conventional production parameters to achieve high output and cost-effectiveness. It is now more crucial than ever to take into account all forms of waste generated during the production process and to take the appropriate steps to prevent, minimise, reuse, or recycle waste that already exists.
2.4.5. Collaborative consumption theory
The collective usage of an item or service by a group is known as collaborative consumption. In contrast to collaborative consumption, which allows several people to have access to a good and share its cost, regular consumption involves a single person paying the entire cost of an item and having exclusive use of it. Flea markets, swap meets, garage sales and second-hand shops are examples of the types of events that have historically given rise to collaborative consumption, which is defined as the practice where consumers both obtain and provide valuable resources or services through direct interaction with peers or through intermediary platforms (Felson & Spaeth, 1978; Belk, 2014). But in recent years, it has attracted more attention and momentum, especially with the introduction of digital technologies like Web 2.0, mobile technology, and social media.
2.4.6. Social sustainability theory
The word “social sustainability,” which has multiple definitions, generally refers to the social aspects of sustainability. Social sustainability theory highlights the need for integrated approaches to sustainable development by acknowledging the interdependence and connectivity of social, economic, and environmental systems. In a normative sense, it refers to the social goals of sustainability initiatives.
2.4.7. Cognitive dissonance theory
It is essential to understand the behaviour of consumers to design strategies regarding the adoption of the circular economy. Several existing theories explain the psychology behind consumer behaviour towards digitalization and circular economy. The first theory is the cognitive dissonance theory which was proposed in 1975 by psychologist Leon Festinger. It provides us insights into consumer behaviour after they have evaluated and used the product or the service and studies their behaviour when they face a discrepancy between the pre-service and post-service perception of the product (Park et al., 2015, Venkatesh and Goyal, 2010). These contradictory cognitions trigger the expectations and psychological state of the user which leads to negative emotions. This can harm the reputation of the brand which can demotivate the individual to adopt the new technology. Previous research shows that consumers experience three emotions namely anger (Harmon-Jones, 2004, Harmon-Jones et al., 2017), guilt (Gosling et al., 2006, Turel, 2016) and regret (Roese and Summerville, 2005, Gilovich et al., 1995b) while experiencing the performance shortcoming.
2.4.8. Unified theory of consumer acceptance
The second theory is the Unified Theory of Consumer Acceptance and Use of Technology which aims to give an understanding of the variables impacting the user behaviour across different contexts and has laid out two key determinants for studying behavioural intention. It is a sub-part of the TAM model. The theory focuses on two main key determinants which are perceived ease of use and perceived usefulness.
2.4.9. ICT theory
The Integration of Information and Communication Technology theory was the result of several theories and past developments. The TAM model and the diffusion of innovations theory have been greatly influential in understanding the behaviour towards ICTs. ICT theory studies the impact and influence of technological developments on communication processes, information, individuals and groups. The theory helps to navigate the complex relationship between the behaviour of individuals and technological tools.
2.4.10. Digital twin theory
Digital twin by definition is the virtual representation of an object. The theory of digital theory revolves around the concept of Industry 4.0 and the transformation of technologies. (IEEE,2020) NASA’s Apollo program had a huge role in the development of this theory as they used digital models for simulations to ensure the reliability of the Apollo missions. It further developed after the emergence of Industry 4.0 where several replicas were created for the systems which helped to improve the overall efficiency of data. (Philips et al., 2020) In recent times the theory has been used due to its operational efficiency and reduced cost. Similarly, this theory can be used in the production of products that hold sustainability. This utilization will lead to better urban planning and use of resources.
2.4.11.Social network theory
Social Network theory examines the social interactions between people, communities and organisations. It looks into various aspects like communication, friendship or resource exchanges. Three main lines of research led to the development of these theories namely sociometric analysis, interpersonal relations and anthropology relations. This framework did not develop till the 1960s and advancements took place with the help of old research studies. In terms of digitalization, it highlights the importance of partnerships and collaborations which can lead to the adoption of a more circular model. The usage of digital tools can also lead to the sharing of ideas and co-creation among people creating a sense of belongingness with one another.
3. RESEARCH METHODS
3.1. Research Instruments
In this inquiry project, research will be conducted through a survey, to be distributed among students aged 20 and above, sustainability-related venture employees and ESG dept employees of various companies. The intention is to try and collect results from a broad cross-section of people and gain valuable insight into variations of perceptions. The survey will comprise a mixture of quantitative and qualitative questions. The quantitative questions will utilise a Likert scale to apply nominal values to responses gauging agreeableness, allowing for a clearer analysis of the data. Each question which requires the respondents to answer on a Likert scale about their perception (i.e., non-definitive questions, such as age) will include a midpoint. This research project will be conducted as a short-term, ‘action research’ (Gray, 2020), this methodology is most suitable as the results are intended to offer action points and promote organisational change if potential improvements are found. While a long-term study could offer an analysis of any change implications, the available timeframe of this project does not allow for this. However, using a short-term, cross-sectional study enables research insight from a broad range of participants.
The results of the survey will first use the analytical tools built into MS Forms, additional analysis will be undertaken by various means, looking to identify correlations between responses. Significant findings will be represented graphically for ease of interpretation in the final report, with discussion drawing attention to them.
3.2. Data Collection
The data collection schedule includes two weeks starting from mid-August, the window has been shortened due to time constraints. To collect data from consumers for this research, questionnaires were distributed online through social media platforms like LinkedIn, X (formally Twitter) etc. and interviews with 5 professionals from sustainability-related industries were conducted. The final questionnaire consisted of eighteen questions in addition to the demographic information. There were 3 questions to collect demographic data like field of occupation, designation, and work experience. A 10-point Likert scale was used,1 being Very Strongly Disagree and 10 being Very Strongly Agree. For analysis, 52 responses were collected and improper responses and outliers were discarded. The demographic results showed that 49% of respondents were students and the rest 51% respondents were middle-level employees. The SmartPLS 4.0 software was used for analysis and structural equational modelling was followed. Structural equational modelling (SEM) is a statistical technique used to analyze between variables using hypothesis testing. SEM was selected as it tests multiple relationships while accounting for measurement error and it can handle latent variables.
3.3. Theoretical Framework
The author has created a theoretical framework using different variables to establish a relationship between them using theories to prove the hypotheses.
Variables:
Independent variable-
1. Collaboration & partnership
2. Optimum waste management
3. Product lifecycle
4. Sustainable design thinking
5. Consumer acceptance
6. Regulatory framework
Sub variables-
1. Silo effect
2. Cloud sharing
3. Shelf life tracking
4. Remanufacturing
5. Automation
6.IOTs (internet of things)
7. Infrastructure for innovation
8. Updated cloud data
9. Greenwashing
10. Price Sensitivity
11. Artificial Intelligence
12. Cloud computing
Dependent variable – Adoption of circular economy in manufacturing
Mediating variable – Data science
Moderating variable- Organizational culture
Outcome variable-
1. Economic benefit
2. Enhanced product lifecycle
3. Extended use of waste material
4. Sustainable living standards
5. Sustainable economic innovation
Hypothesises-
H1:Collaboration and partnership help data science for the adoption of circular economy in manufacturing
H2:Consumer Acceptance helps data science for the adoption of circular economy in manufacturing
H3:Data Science in Adoption of circular economy in manufacturing positively impacts economic benefit
H4: Data Science in Adoption of circular economy in manufacturing positively impacts enhanced product lifecycle
H5: Data Science in the Adoption of circular economy in manufacturing positively impacts the extended use of waste material
H6: Data Science in Adoption of circular economy in manufacturing positively impacts sustainable economic innovation
H7: Data Science in Adoption of circular economy in manufacturing positively impacts sustainable living standards
H8: Optimum waste management helps data science for the adoption of a circular economy in manufacturing
H9:Product lifecycle helps data science for the adoption of circular economy in manufacturing
H10: Regulatory framework helps data science for the adoption of circular economy in manufacturing
H11: Sustainable design thinking helps data science for the adoption of circular economy in manufacturing
4. Research Design and Methods
4.1. Project Design
The inquiry will be conducted primarily by a questionnaire distributed to target respondents. This will be distributed, utilising various social media means. The aim of this is to allow for a diverse range of respondents. The decision to distribute the survey should remove noncoverage errors, though there is a risk of nonresponse errors occurring (Sills & Song, 2002). Further to allowing this first level of privacy where possible an option for randomised responses will be utilised to combat evasive answer bias (Warner, 1965).
Distributing the survey online presents a risk of non-response, with electronic surveys shown to record lower response rates than physical means of distribution (Nayak & Narayan, 2019). (Dilman, et al. 2009) showed that the highest response rates were to incentivised surveys, it was decided that offering a monetary incentive to complete the survey was not suitable for this inquiry project.
Much of the survey will provide quantitative data to analyse, through the use of Likert scale questions. Using a Likert scale allows the conversion of ordinal data to interval data, either directly or by use of composite scoring (sums and averages) (Willits, Theodore, & Luloff, 2016), this allows quick calculation of average responses and simple plotting of correlated data sets to graphs. Care will be taken to consider missing information when calculating averages. When analysing Likert scale responses mean values will be used to show central tendencies, but these may produce an excess of neutral results, if this is the case standard deviations can be used to express the variability of results (Boone & Boone, 2012). Other than that P values, Cronbach alpha values and AVE will be used for reliability and validity Testing along with hypothesis testing. The structure of interviews defines the qualitative analysis. Semi-structured interviews have been used and for analysis, the outcomes of each interview have been divided into common, contradicting and different views and later these are used along with the quantitative results to prove the hypotheses.
Multiple response methods are being used to produce a more accurate picture of people’s beliefs and feelings, offering holistic and demonstrable action points. Having to analyse and synthesise the findings of both quantitative and qualitative data will take longer, however, the benefit of a more complete insight far outweighs the additional time and effort (Shah & Corley, 2006).
4.2. Timescale and Resources
It is intended that this research inquiry be completed in 10 weeks, with the final report available at the end of August 2022.
The literature review was conducted in the first week, with findings used to inform the survey structure and lines of question. The aim is for all approvals and data collection schedules to be completed by the fourth week to maximise the available time for analysis. The final week of the inquiry project will be composing a full and final report document, with review and scrutiny of results. This review and confirmation of result validity will be the final stage of the research to verify the accurate reporting of the findings
It is not expected that any additional resources will be required, other than the time of respondents to complete the survey, this is expected to not be any more than 10 minutes per respondent. Initial response numbers were considered insufficient for analysis, which is why there was a need for semi-structured interviews to aid in the quality of results through qualitative analysis. Analysis has been completed as a sole endeavour, providing a singular perspective on the results of the survey and reducing the chance of compromising the anonymity of responses.
5. Data Analysis
The first four questions in the survey were the only mandatory responses in the survey, as they and the participant information sheet formed the consent for respondents, as such all completed surveys returned yes responses. The demographic shows 49% of respondents as students and 51% as middle-level employees.

Next questions were composed using a Likert scale, all with responses 1-10, the wording associated with the high and low scores was tailored to suit each question, maintaining like phrases where possible. The common approach of a ten-point scale was maintained for continuity and fair comparison and to ensure the tangible validity of results.
Regarding all the survey questions we set the positive benchmark as 8 out of 10 and the lowest average achieved was 8.54 out of 10 which strongly proved the likeliness of the proposed hypothesis. With an overall average of 9.05 out of 10, there was no problem in proving the likeliness of the hypothesises. The hypothesis whose likeliness is proved in the weakest was consumer acceptance helps data science for the adoption of circular economy in manufacturing. It had 17.30% of responses did not support the claim or hypothesis. This was the highest percentage for a non-supporting population in the whole analysis but as we can see the values are still acceptable as the likeliness score percentage is above 80 I.e.82%.

Now to strengthen the claims and hypotheses, the author used SmartPLS4.0 to establish relationships and get deeper analytic statistics.
The reliability was assessed by using Cronbach’s alpha value as shown below. The respective values of Cronbach alpha for all the variables were much higher than 0.7 which led to considerable acceptance of all the values. For determining model reliability, Cronbach’s alpha was used where all the values were greater than 0.7 respectively. (Cronbach, 1951).
Cronbach’s alpha | |
Collaboration & Partnership | 0.973 |
Consumer acceptance | 0.948 |
Economic benefit | 0.933 |
Enhanced product lifecycle | 0.949 |
Extended use of waste material | 0.941 |
Optimum waste management | 0.949 |
Product Lifecycle | 0.96 |
Regulatory framework | 0.902 |
Sustainable design thinking | 0.947 |
Sustainable economic innovation | 0.973 |
Sustainable living standard | 0.948 |
Next, the validity was tested using AVE, where the acceptable value was set to AVE > 0.5 and all the values were found to be acceptable as shown below, thus proving the validity of the data.
Average variance extracted (AVE) | |
Collaboration & Partnership | 0.973 |
Consumer acceptance | 0.951 |
Economic benefit | 0.937 |
Enhanced product lifecycle | 0.952 |
Extended use of waste material | 0.945 |
Optimum waste management | 0.952 |
Product Lifecycle | 0.961 |
Regulatory framework | 0.91 |
Sustainable design thinking | 0.95 |
Sustainable economic innovation | 0.973 |
Sustainable living standard | 0.951 |
Now to strongly prove all the hypothesises, the P value was taken into consideration. The hypothesis was tested through a P-test (Armstrong & Overton, 1977) where respondents with missing responses were considered non-respondents. (Kam & Meyer, 2015)It was found that all the P values were below 0.05 and hence all were strongly supported and proved all the hypotheses true.
P VALUES | |
Collaboration & Partnership -> Data Science | 0.003 |
Consumer acceptance -> Data Science | 0.007 |
Data Science -> Economic benefit | 0.000 |
Data Science -> Enhanced product lifecycle | 0.000 |
Data Science -> Extended use of waste material | 0.000 |
Data Science -> Sustainable economic innovation | 0.000 |
Data Science -> Sustainable living standard | 0.000 |
Optimum waste management -> Data Science | 0.001 |
Product Lifecycle -> Data Science | 0.002 |
Regulatory framework -> Data Science | 0.000 |
Sustainable design thinking -> Data Science | 0.002 |
5.1.Findings
H1:Collaboration and partnership help data science for the adoption of circular economy in manufacturing was highly supported ( p <0.05)
Transition to circular economies is often troublesome due to differences in objectives. The majority of the time, stakeholders face many conflicts due to each other’s different priorities and objectives. Accordingly, cooperation is regarded as a crucial key for unlocking everything that circular economies have to offer a business. Transition mostly stops due to overlying costs of implementation that stakeholders do not want to face They fail to see the broad picture or the betterment of the planet. Partnerships come into play here. Also, public government partnerships can contribute to the collection of waste and recycling rates. Overall, valuable partnerships and collaborations with the government and private institutions with the help of data science can lead to easier adoption of circular economy in manufacturing.
H2:Consumer Acceptance helps data science for the adoption of circular economy in manufacturing was highly supported ( p <0.05)
It has been highlighted that low consumer acceptance is a major boon in case one wants to scale one’s business models (CBMs) towards circular economies. Digitalisation urges innovative ways to exchange and share products and certain services. Technologies such as big data and IoT keep inventory checks and aid in utilising underused products and assets. Furthermore, organisations leverage these technologies such as digital platforms, and augmented reality for collecting advanced product imagery to address obstacles with security, convenience, trust and hygiene. They increase consumer acceptance with increased transparency and remove quality and authenticity concerns. Thus, waste reduction and up-cycling of by-products are made possible by an increment in consumer understanding of circular economy principles. Consumers can be made more aware through trust-building, addressing potential risks, and spreading awareness.
H3:Data Science in the Adoption of circular economy in manufacturing positively impacts economic benefit was highly supported ( p <0.05)
Manufacturers can save operational costs by reducing waste, optimising resource utilisation, and identifying inefficiencies by analysing massive datasets. Predictive maintenance and lifecycle analysis are further made possible by data science, which increases product longevity and lowers the requirement for raw materials. In addition to reducing expenses, this also creates new opportunities for profit through remanufacturing, recycling, and cutting-edge business concepts like product-as-a-service. All things considered, applying data science to circular economy strategies increases revenue while advancing sustainability.
H4: Data Science in the Adoption of circular economy in manufacturing positively impacts enhanced product lifecycle was highly supported ( p <0.05)
Manufacturers may create more robust and repairable products by using advanced analytics to obtain insights into product usage, wear, and failure trends. With the use of this data-driven method, a product’s lifecycle can be precisely monitored, allowing for timely maintenance and upgrades that increase the product’s lifespan. Additionally, data science ensures that resources are continuously reused, enabling the circular economy, by optimising material recycling and repurposing at the end of a product’s lifecycle.
H5: Data Science in the Adoption of circular economy in manufacturing positively impacts extended use of waste material was highly supported ( p <0.05)
Data science finds ways to recover and reuse materials that would otherwise be thrown away by examining waste streams and material flows. This lowers the requirement for virgin resources by enabling producers to turn trash into useful inputs for new goods. Additionally, by ensuring that materials are effectively sorted, processed, and reintegrated into production cycles, predictive analytics aids in the optimisation of the recycling process. This expanded utilisation of waste not only promotes sustainability but also lowers expenses and improves manufacturing resource efficiency.
H6: Data Science in the Adoption of circular economy in manufacturing positively impacts sustainable economic innovation was highly supported ( p <0.05)
We can develop novel approaches to creating goods that are intrinsically sustainable—designed for disassembly, repair, and reuse—by examining patterns of material consumption, waste production, and product lifecycles. Additionally, data science opens the door for creative economic models that reduce waste and repurpose it as a resource, like closed-loop systems and circular supply chains. With this strategy, sustainability becomes a source of economic value and changes the way we see consumption and production. We can test and scale these advances with data science’s rigorous analysis and iterative process, guaranteeing that environmental stewardship and economic growth are mutually reinforcing rather than conflicting.
H7: Data Science in the Adoption of circular economy in manufacturing positively impacts sustainable living standards was highly supported ( p <0.05)
Manufacturers may use data-driven insights to optimise resource utilisation, reduce waste, and build products that are more durable, efficient, and environmentally friendly. This transition not only minimises the environmental footprint but also makes sustainable products more accessible and inexpensive, hence increasing consumers’ quality of life. Furthermore, data science enables the creation of innovative business models that prioritise sustainability, such as product leasing or take-back programs, which help to integrate circular economy ideas into everyday life. As a result, integrating data science into manufacturing contributes to a more sustainable future in which economic growth and higher living standards coexist with environmental care.
H8: Optimum waste management helps data science for adoption of circular economy in manufacturing was highly supported ( p <0.05)
The implementation of the circular economy in manufacturing can be supported by data science to a greater extent through optimal waste management. Data science can track and analyse waste streams, spot trends, and locate opportunities for resource recovery and recycling by managing trash effectively. In line with the circular economy’s tenets, producers can minimise waste, recycle materials, and develop closed-loop systems with the aid of this data-driven strategy. In the end, efficient waste management offers the information and understanding needed to create more environmentally friendly production processes that also maximise resource efficiency.
H9:Product lifecycle helps data science for the adoption of circular economy in manufacturing was highly supported ( p <0.05)
The product life cycle emphasises sustainable practices and optimization of resources. It directly influences the adoption of a circular economy. The alteration from traditional linear models to circular economy practices comprises reusing, repairing, upgrading and recycling goods throughout their life cycle to mitigate waste generation, consumption of resources and impact on the environment. Additionally, including circularity indicators by using IOTs and cloud computing throughout the design, manufacturing, distribution and use phases of products is important for improving and measuring circular economy performance in sectors like electrical and electronic manufacturing with data science elements.
H10: Regulatory framework helps data science for adoption of circular economy in manufacturing was highly supported ( p <0.05)
Regulatory Framework is an important factor in the implementation of the circular economy. The adoption of a circular economy is driven by Government policies and initiatives in various industries. The European Union has also adopted regulations and guidelines, such as the Circular Economy Package, to encourage circularity in various industries and provide a legal framework that facilitates the shift towards sustainability. Furthermore, the implementation of guidelines such as the Regulation of Taxonomy for sustainable operations aims to establish a standard language for investors, providing incentives for environmentally beneficial initiatives and encouraging businesses to implement circular economy principles. These regulatory measures would not only help in guiding companies but also provide incentives to drive towards a more sustainable future and with cloud structures and AI, it will be easy to track the implementation of regulatory frameworks.
H11: Sustainable design thinking helps data science for adoption of circular economy in manufacturing was highly supported ( p <0.05)
Sustainable design thinking integrates circular economy principles with data science to transform manufacturing processes. By using data-driven insights, manufacturers can identify inefficiencies, optimize resource use, and minimize waste. Sustainable design thinking guides these efforts, ensuring that solutions not only improve operational efficiency but also align with circular economy goals, such as reducing waste, promoting material reuse, and extending product life cycles. This synergy between data science and sustainable design creates a more resilient, eco-friendly manufacturing system
6. Conclusions
6.1. Introduction
In this concluding chapter the findings will be summarised according to the research objectives, followed by a short section on the contribution to knowledge. Then the limitations of the study will be discussed. Finally, recommendations with implications for theory, further research and practice are presented.
6.2. Summary of findings
This study aimed to achieve the following:
- To gain insights and explore the scope of the circular economy.
- To identify and investigate how data science impacts the circular economy.
- To establish a relationship between data science and circular economy in manufacturing
- To observe the influence of data science in circular economy in manufacturing
- To gain insights and explore the scope of the circular economy.
We concluded how important and relevant circular economy is as by shifting from a traditional linear model of production and consumption to a circular approach, businesses and societies can reduce waste, optimize resource use, and create new economic opportunities. This model emphasizes the importance of designing for longevity, repairability, and recyclability, ultimately contributing to environmental conservation and economic resilience. As we continue to investigate and implement circular economy principles, it becomes increasingly evident that such strategies are essential for addressing the pressing challenges of resource depletion and climate change.
- To identify and investigate how data science impacts the circular economy
In conclusion, identifying and investigating how data science impacts the circular economy revealed its pivotal role in enhancing resource efficiency and sustainability. Data science enables more precise tracking and analysis of resource flows, product lifecycles, and waste management processes, leading to optimized circular practices. By leveraging advanced analytics, machine learning, and predictive modelling, businesses can improve decision-making, forecast demand, and identify opportunities for recycling and reuse. This data-driven approach not only supports the transition to a circular economy but also drives innovation and operational efficiency
- To establish a relationship between data science and circular economy in manufacturing
In conclusion, we got to know how collaboration & partnership, Optimum waste management, product lifecycle, sustainable design thinking consumer acceptance and regulatory framework play a pivotal role in helping data science in the adoption of a circular economy in manufacturing.
- To observe the influence of data science in circular economy in manufacturing
In conclusion, we got to know that data science in circular economy in manufacturing leads to economic benefit, enhanced product lifecycle, extended use of waste material, sustainable living standards and sustainable economic innovation
6.3. Limitations
As with any study, this research suffers from limitations. The most important ones are mentioned ahead
Firstly, the existing literature is sparse. Manufacturing-specific literature was hard to find. Available literature lacked narrowing down the topic of the circular economy into the manufacturing sector and then linking it up with data science. Additionally, the small sample size might have biased the results due to the fact, that the data collection through simple random sampling failed to achieve the desired sample size of 384 respondents (Biggam, 2017, 171 and Saunders et al., 2019, 302) during the data collection period. Instead, only 52 cases could be collected and analyzed. Hence, the sample might not reflect the total population. A small sample size also increases the error margin resulting in less precise conclusions on the population.
6.4. Recommendation
The findings and limitations of this study have been used to develop recommendations with implications for theory, further research and practice.
6.4.1. Implications for theory and further research
- Increase the sample size in further research
A larger sample size of 384 respondents is recommended for further research. This ensures the representativeness of the evidence gathered regarding the population as a confidence level of 95% is reached, subsequently reducing the error margin to 5% (Biggam, 2017, 171 Saunders et al., 2019, 302).
- Extend the data collection period in further research
In the current study, the data collection was limited to two weeks, partly in the summer holiday period. An extension to a period of one month would reduce the non-response rate due to the absence or busy schedule of the respondents.
REFERENCES
Bressanelli, G., Saccani, N., & Perona, M. (2022, January 6). Investigating Business Potential and Users’ Acceptance of Circular Economy: A Survey and an Evaluation Model. Sustainability. https://doi.org/10.3390/su14020609
Camacho-Otero, J., Boks, C., & Pettersen, I. N. (2018, August 4). Consumption in the Circular Economy: A Literature Review. Sustainability. https://doi.org/10.3390/su10082758
Kulviwat, S., Bruner, G. C., Kumar, A., Nasco, S. A., & Clark, T. (2007, November 7). Toward a unified theory of consumer acceptance technology. Psychology & Marketing. https://doi.org/10.1002/mar.20196
Marikyan, D., Papagiannidis, S., & Alamanos, E. (2020, July 25). Cognitive Dissonance in Technology Adoption: A Study of Smart Home Users. Information Systems Frontiers. https://doi.org/10.1007/s10796-020-10042-3
Wen, H., Lee, C., & Song, Z. (2021, May 20). Digitalization and environment: how does ICT affect enterprise environmental performance? Environmental Science and Pollution Research. https://doi.org/10.1007/s11356-021-14474-5
The Digital Twin Concept in Industry – A Review and Systematization. (2020, September 1). IEEE Conference Publication | IEEE Xplore. https://ieeexplore.ieee.org/abstract/document/9212089
Fleischmann, M., Bloemhof-Ruwaard, J. M., & Dekker, R. (2000). Logistics Impact on Inventory Management. International Journal of Physical Distribution & Logistics Management, 30(3/4), 282-305.
Tesla. (2022). Tesla, Inc. 2021 Impact Report.
IKEA. (2022). IKEA Sustainability Report FY21.
Womack, J. P., Jones, D. T., & Roos, D. (1990). The Machine That Changed the World: The Story of Lean Production. Simon and Schuster.
Harry, Schroeder, R. (2000). Six Sigma: The Breakthrough Management Strategy Revolutionizing the World’s Top Corporations. Currency.
Signify. (2021). Sustainability Annual Report 2020.
Picot, A., Reichwald, R., & Wigand, R. T. (2008). Information, Organization and Management: Expanding Markets and Corporate Boundaries. Springer Science & Business Media.
Top of Form
European Commission. (2020). A new Circular Economy Action Plan for a cleaner and more competitive Europe. Retrieved from https://ec.europa.eu/environment/circular-economy/pdf/new_circular_economy_action_plan.pdf
European Parliament. (2019). EU legislation is in place for the circular economy. Retrieved from https://www.europarl.europa.eu/RegData/etudes/IDAN/2019/631060/EPRS_IDA(2019)631060_EN.pdf
Philips. (n.d.). Digital Twin Technology for Healthcare. Retrieved from https://www.philips.com/a-w/research/connected-care-and-health-solutions.html
Walter Fraanje a b, a, b, AbstractCollaborative consumption indicates the emergence and rapid spread of a new set of consumption practices. Originally praised as an antidote to an unsustainable market economy, Belk, R., Cheng, M., … MyWheels, n. d. (2018). What future for collaborative consumption? A practice theoretical account. Retrieved from https://www.sciencedirect.com/science/article/abs/pii/S0959652618329305
Data Silos, why they’re a problem, & how to fix it. (n.d.). Retrieved from https://www.talend.com/resources/what-are-data-silos/
T, K. (2023a). What does a memorandum of understanding accomplish? Retrieved from https://vakilsearch.com/blog/what-does-memorandum-of-understanding-accomplish/
Vadén, T., Lähde, V., Majava, A., Järvensivu, P., Toivanen, T., Hakala, E., & Eronen, J. T. (2020, October 1). Decoupling for ecological sustainability: A categorisation and review of research literature. Environmental Science & Policy. https://doi.org/10.1016/j.envsci.2020.06.016
Figure 1. Relative and absolute decoupling (modified from UNEP, 2011). (n.d.). ResearchGate. https://www.researchgate.net/figure/Relative-and-absolute-decoupling-modified-from-UNEP-2011_fig1_331234240
Resource efficiency and the circular economy: concepts, economic benefits, barriers, and policies – UCL Discovery. (n.d.). https://discovery.ucl.ac.uk/id/eprint/10054117/
Van Ewijk, S. (2018, January 1). Resource efficiency and the circular economy: Concepts, economic benefits, barriers, and policies. ResearchGate. https://www.researchgate.net/publication/327868697_Resource_efficiency_and_the_circular_economy_Concepts_economic_benefits_barriers_and_policies
Winkler, H. (2011, January 1). Closed-loop production systems—A sustainable supply chain approach. CIRP Journal of Manufacturing Science and Technology. https://doi.org/10.1016/j.cirpj.2011.05.001
Chen, J. (2023, January 30). Collaborative Consumption: What it is, How it Works. Investopedia. https://www.investopedia.com/terms/c/collaborative-consumption.asp
National Environment Agency. (2020). Singapore Circular Economy Roadmap. Retrieved from https://www.nea.gov.sg/programmes-grants/circular-economy/singapore-circular-economy-roadmap
Wasserman, S., & Faust, K. (1994). Social Network Analysis: Methods and Applications. Cambridge University Press.
Camacho-Otero, J., Tunn, V., Chamberlin, L., & Boks, C. (2020, December 15). Consumers in the circular economy. Edward Elgar Publishing eBooks. https://doi.org/10.4337/9781788972727.00014
Partridge, E. (2014, January 1). Social Sustainability. Springer eBooks. https://doi.org/10.1007/978-94-007-0753-5_2790
Milios, L. (2021, January 21). Towards a Circular Economy Taxation Framework: Expectations and Challenges of Implementation. Circular Economy and Sustainability, 1(2), 477–498. https://doi.org/10.1007/s43615-020-00002-z
Kirchherr, J., Yang, N. H. N., Schulze-Spüntrup, F., Heerink, M. J., & Hartley, K. (2023, July 1). Conceptualizing the Circular Economy (Revisited): An Analysis of 221 Definitions. Resources, Conservation and Recycling. https://doi.org/10.1016/j.resconrec.2023.107001
Morseletto, P. (2020, February 1). Targets for a circular economy. Resources, Conservation and Recycling. https://doi.org/10.1016/j.resconrec.2019.104553
Kirchherr, J., Reike, D., & Hekkert, M. P. (2017, December 1). Conceptualizing the circular economy: An analysis of 114 definitions. Resources, Conservation and Recycling. https://doi.org/10.1016/j.resconrec.2017.09.005
A Model for the Transition to the Circular Economy: The “R” Framework. Symphony, doi: 10.4468/2022.1.08fornasari.neri
A., Dwivedi., Sanjoy, K., Paul. (2022). A framework for digital supply chains in the era of circular economy: Implications on environmental sustainability. Business Strategy and The Environment, doi: 10.1002/bse.2953
Jinghua, Liu., Muhammad, Umer, Quddoos., Muhammad, Hanif, Akhtar., Muhammad, Sajid, Amin., Muhammad, Junaid, Tariq., Arij, Lamar. (2022). Digital technologies and circular economy in supply chain management: in the era of COVID-19 pandemic. Operations Management Research, doi: 10.1007/s12063-021-00227-7
Pietro, De, Giovanni. (2023). The Impact of Digital Technologies and Sustainable Practices on Circular Supply Chain Management. Logistics doi: 10.3390/logistics7010001
Angelina, Pavlović., Snežana, Nestić., Goran, Bošković. (2021). Circular economy management in business organizations using digital technologies. doi: 10.5937/SJEM2101022P
Fiona, Charnley., Fabienne, Knecht., Helge, Muenkel., Diana, Pletosu., Victoria, A., Rickard., Chiara, Sambonet., Martina, Schneider., Chunli, Zhang. (2022). Can Digital Technologies Increase Consumer Acceptance of Circular Business Models? The Case of Second Hand Fashion. Sustainability, doi: 10.3390/su14084589
Petros, Spachos., Weiwei, Li., Mark, Chignell., Alberto, Leon-Garcia., Leon, Zucherman., Jie, Jiang. (2015). Acceptability and Quality of Experience in over-the-top video. doi: 10.1109/ICCW.2015.7247424
Boriana, Rukanova., Jolien, Ubacht., Ben, Turner., Yao-Hua, Tan., Jonathan, Schmid., E., Rietveld., Wout, Hofman. (2023). A Framework for Understanding Circular Economy Monitoring: Insights from the Automotive Industry. doi: 10.1145/3598469.3598530
Tommaso, Fornasari., Neri, Paolo. (2022).
Fiona, Charnley., Fabienne, Knecht., Helge, Muenkel., Diana, Pletosu., Victoria, A., Rickard., Chiara, Sambonet., Martina, Schneider., Chunli, Zhang. (2022). Can Digital Technologies Increase Consumer Acceptance of Circular Business Models? The Case of Second Hand Fashion. Sustainability, doi: 10.3390/su14084589
Miroslav, Jurkovič. (2022). How to Increase Consumer Acceptance of the Transition to Circular Economy. Conference proceedings, doi: 10.53465/edamba.2021.9788022549301.226-233
Anindita, Prabawati., Evi, Frimawaty., Joko, Tri, Haryanto. (2023). Strengthening Stakeholder Partnership in Plastics Waste Management Based on Circular Economy Paradigm. Sustainability, doi: 10.3390/su15054278
P., Giovani, Palafox-Alcantar., Dexter, V., L., Hunt., Christopher, D., F., Rogers. (2021). Current and future professional insights on cooperation towards circular economy adoption. Sustainability, doi: 10.3390/SU131810436
Sepani, Senaratne., KC, Abhishek., Srinath, Perera., Laura, M., M., C., E., Almeida. (2021). Promoting stakeholder collaboration in adopting circular economy principles for sustainable construction. doi: 10.31705/WCS.2021.41
Foivos, Psarommatis., Gökan, May. (2022). Achieving Global Sustainability Through Sustainable Product Life Cycle. doi: 10.1007/978-3-031-16407-1_46
Olga, Timofei. (2023). Circular business models for increasing product usage. doi: 10.53486/cike2022.08
Kam, C. C. S., & Meyer, J. P. (2015). How careless responding and acquiescence response bias can influence construct dimensionality: The case of job satisfaction.
Armstrong, J. S., & Overton, T. S. (1977). Estimating nonresponse bias in mail surveys. Journal of Marketing Research, 14(3), 396–402.
B., M., Hapuwatte.. (2023). Optimizing Product Life Cycle Systems for Manufacturing in a Circular Economy. doi: 10.1007/978-3-031-28839-5_47
Cronbach, L. J. (1951). Coefficient alpha and the internal structure of tests. Psychometrika, 16(3), 297–334. https://doi.org/10.1007/BF02310555.
Patwa, N., Sivarajah, U., Seetharaman, A., Sarkar, S., Maiti, K., & Hingorani, K. (2021, January 1). Towards a circular economy: An emerging economies context. Journal of Business Research. https://doi.org/10.1016/j.jbusres.2020.05.015
About the author
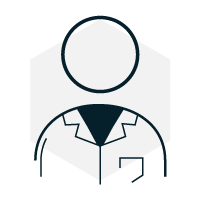
Ritam Bhandari
Ritam is a motivated individual who is really interested in the field of sustainability.