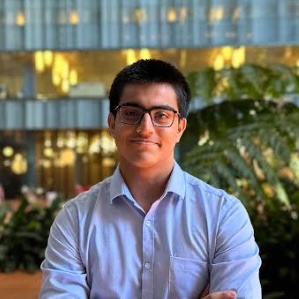
Author: Vedanth Ramji
Mentor: Mr. Arun Moses
APL Global School, Chennai, India
Abstract
Motivation: Wastewater treatment plants (WWTPs) are known to be unable to completely remove antimicrobial resistance genes (ARGs) and antimicrobial-resistant bacteria (ARBs). This causes the contamination of freshwater environments with ARGs and ARBs. Most surveillance efforts to monitor antimicrobial resistance (AMR) in wastewater treatment plants currently occurs at the scale of single treatment plants. However, global surveillance of AMR in different WWTPs is crucial to develop a better understanding of the global WWTP resistome. Culture-independent metagenomics approaches are increasingly being used to monitor AMR in the environment, especially in wastewater. This study leverages publicly available metagenomes of WWTP influent and effluent to compare the impact of WWTPs on microbial diversity of resistant pathogens and distributions of ARGs and ARBs globally.
Results: A similar distribution and frequency of resistant pathogens and ARGs in global influent and effluent was found. ARGs conferring resistance to clinically crucial drug classes were also found in high frequencies in global WWTP influent and effluent.
1. Introduction
Antimicrobial resistance (AMR) is a major environmental and public health concern [1]. AMR occurs when microorganisms change in ways which cause them to withstand antimicrobial drugs, rendering them ineffective, due to environmental pressure. It has been estimated that by 2050, AMR could cause more than 10 million deaths per year [1, 2], causing more loss of life than cancer, diabetes, and diarrheal disease [1]. Furthermore, AMR is an environmental threat as it has been shown to reduce the diversity and abundance of microbial communities in soil that contribute to ecosystem balance [3] and increase morbidity and mortality rates in animals [4].
AMR is mediated by factors such as the overuse of antimicrobials, mutations, mobile genetic elements, and horizontal gene transfer [5 – 7], which are spread through the environment in wastewater, soil, manure applications, and direct exchange between humans and animals [8]. Aquatic environments have been identified as crucial transmission routes for AMR [9]. Globally, antibiotic resistant bacteria (ARBs) and antimicrobial resistance genes (ARGs) enter waterways through effluents from hospitals, agriculture, aquaculture, and pharmaceutical activity [10 – 15]. Antibiotics residues (AR), ARBs, and ARGs are now starting to be recognized as a new category of water contaminants due to their adverse effects on aquatic ecosystems, the environment, and human health [12, 26].
Wastewater treatment plants (WWTPs) are known to be unable to completely remove antimicrobial resistance genes (ARGs) [12-14] and can contaminate freshwater environments [10, 12]. Currently, the surveillance of AMR in the environment continues to lag behind other efforts to curtail AMR [16]. Though studies have been conducted to monitor AMR dissemination by particular WWTPs [17-20], the current research does not focus on global monitoring of AMR in WWTPs [21]. Global monitoring of AMR dissemination by WWTPs is crucial to understanding AMR in the environment and tackling it using an integrated ‘One Health’ approach which balances and optimizes the health of the environment, animals and humans [21].
Metagenomics is the study of genomic data derived from environmental and clinical samples by sequence analysis or functional gene screening [22, 23]. Metagenomics is culture-independent [24] and hence can be used to compare the distributions of antimicrobial resistance genes and pathogenic microbial species in vast wastewater influent and wastewater effluent samples. Chan Zuckerberg ID (CZ ID) is a cloud-based genomic analysis platform that enables the detection of microbes in metagenomic data and identification of ARGs [25].
This study aims to investigate dissemination of AMR in WWTPs globally by comparing the frequencies and distributions of ARGs and pathogenic microbial species in freely-available metagenomes sourced online through the metagenomic next-generation sequencing (mNGS) and AMR pipelines of CZ ID [25].
2. Materials and Methods
2.1. Sourcing metagenomic data from WWTP influent and effluent samples
The European Nucleotide Archive (ENA) [27] and NCBI’s Sequence Read Archive [28] were both used to source WWTP influent and effluent metagenomes from Italy, South Korea, and Denmark. Table 1 below summarizes the country of origin, sample type and SRA run numbers of the samples. The metagenomes were downloaded as fastq files.
Country | Sample Type | SRA Run Number |
Italy | WWTP Influent | SRR17409990 |
Italy | WWTP Effluent | SRR17409993 |
South Korea | WWTP Influent | SRR11536956 |
South Korea | WWTP Effluent | SRR11536955 |
Denmark | WWTP Influent | SRR28714991 |
Denmark | WWTP Effluent | SRR28714992 |
2.2. Running influent and effluent metagenomes through the CZ ID mNGS and AMR pipelines
Once fastq files for WWTP influent and effluent metagenomes were obtained, they were uploaded to the mNGS Illumina and AMR pipelines of CZ ID. The mNGS Illumina pipeline (Figure 1) is designed to identify microbes within genomic sequences from mixed microbial communities using BLASTN and BLASTX which are algorithms to compare DNA sequences to reference databases [29]. The AMR pipeline (Figure 2) reports bacterial AMR sequences in the Comprehensive Antibiotic Resistance Database (CARD) from genomes and metagenomes using the Resistance Gene Identifier (RGI) [30]. The CARD is a database of molecular determinants of AMR that leverages an ontology graph structure (the Antibiotic Resistance Ontology – ARO) with ARG sequences and mutation data [30]. RGI is an algorithm developed by the CARD team for computational AMR genotype and phenotype prediction using the CARD database [30]. A detailed pipeline visualization of CZ ID can be found in Figure 3.



3. Results and Discussion
3.1. Distributions of pathogenic microbes in global WWTP influent and effluent samples are similar with a slight increase in pathogenic microbes in effluent
A heatmap of known pathogen species from global WWTP influent and effluent metagenomes was generated using the mNGS output of CZ ID (Figure 5). A threshold of NT rPM >= 15 was set to ensure reported species were found with significant relative abundances. NT rPM refers to the number of metagenomic reads aligning to a taxon in the NCBI nucleotide (NT) database per million reads sequenced and is used to compare relative abundance of species across samples. NT rPM can be calculated using the formula shown below, where ERCC refers to the External RNA Controls Consortium spike-in controls which are synthetic RNA molecules used to assess the performance of RNA sequencing experiments [31].

Paired samples t-tests were then performed using the SciPy Python library [32] on the NT rPM of all pathogen species detected for WWTP influent and effluent samples of each country to determine the significance of difference in microbial composition between influent and effluent samples (Table 2). Code to perform the t-tests can be found in Figure 4.
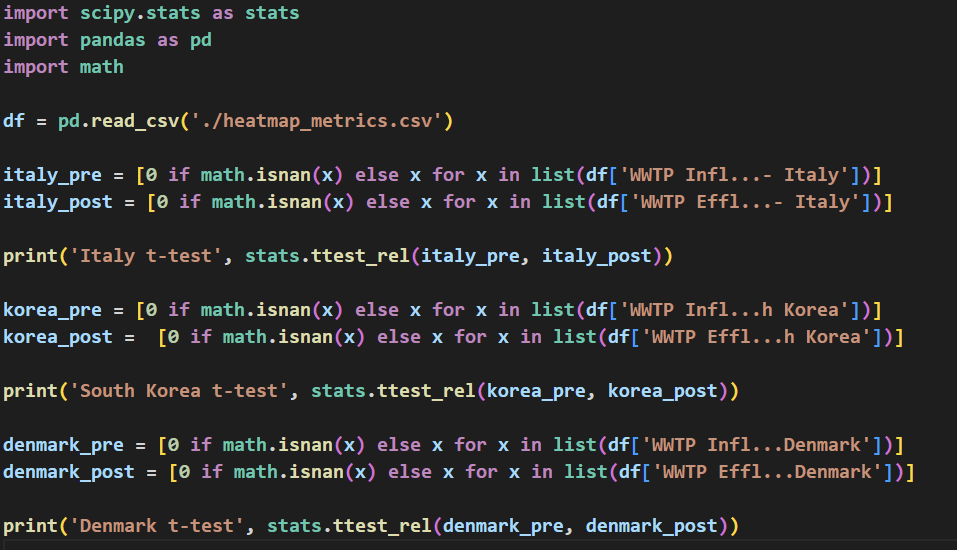
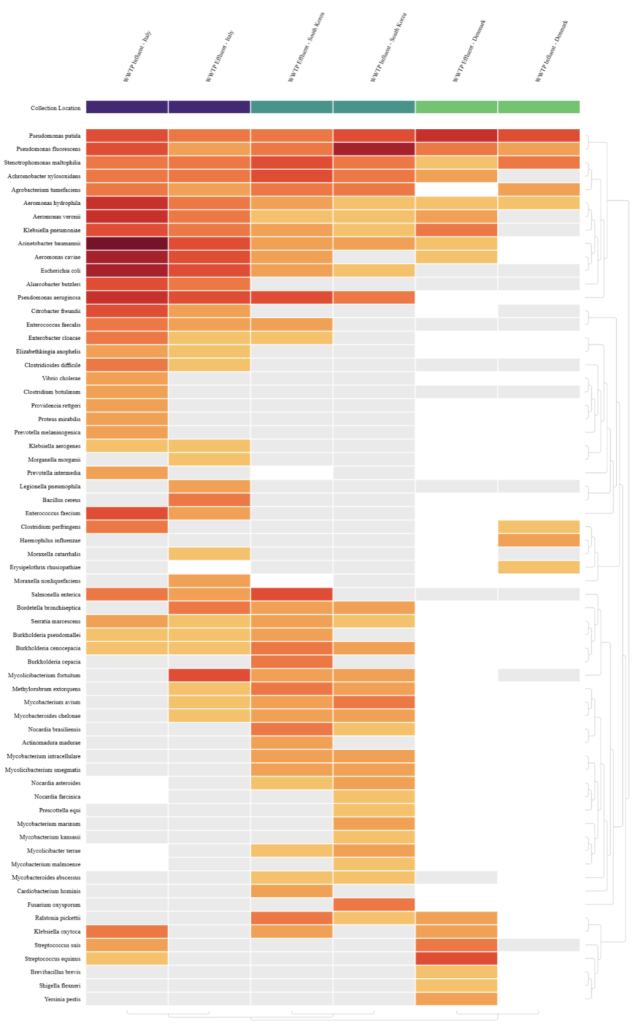
Country | t-test result |
Italy | 1.97 |
South Korea | 0.35 |
Denmark | -2.26 |
A significant difference between WWTP influent and effluent samples was not found for the treatment plants from Italy and South Korea with both samples having a t-test value lesser than 2 (1.97 for Italy and 0.35 for South Korea). This shows that WWTPs were unable to help remove pathogenic microbes from influent. This is supported by Waśko et al, who showed that WWTPs could not remove β-lactam resistance genes from influent and in some cases even increased β-lactam ARGs in effluent [33]. The significant negative t-test result for WWTP influent and effluent from Denmark with a magnitude greater than 2 (-2.26) also supports the findings of Waśko et al, showing an increase in pathogenic species in the effluent sample compared to the influent.
3.2. Frequencies of AMR gene families in global WWTP influent and effluent samples are similar and there are unique gene families in effluent samples
AMR gene families of global influent and effluent samples were combined and their frequencies were plotted on a stacked bar graph (Figure 6).
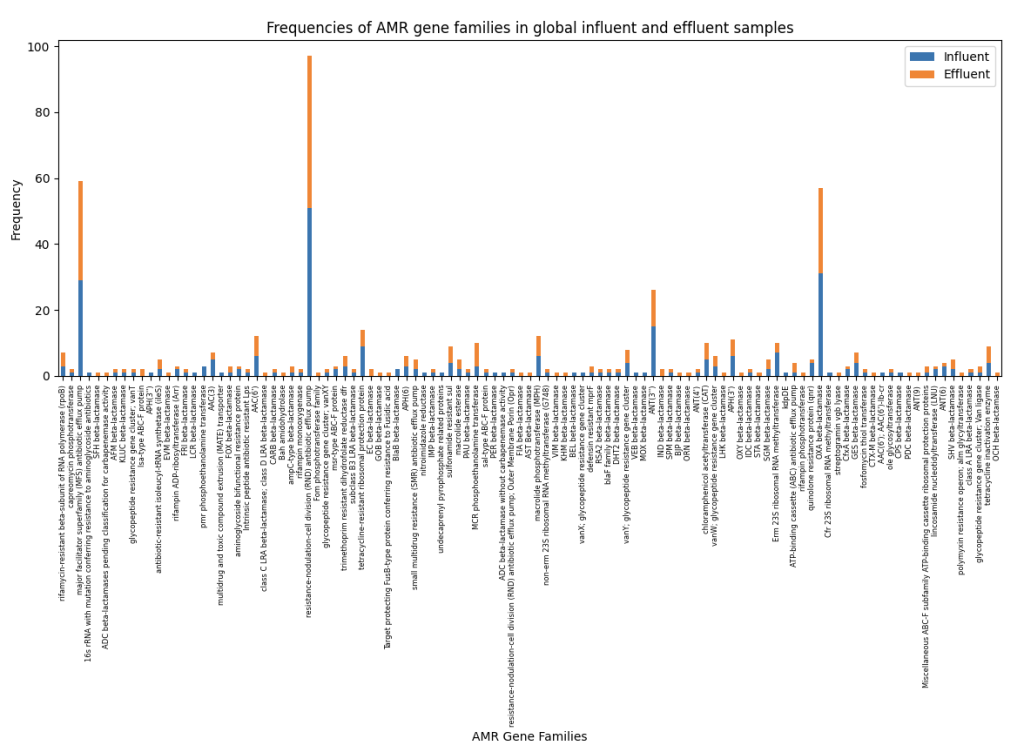
Figure 6 shows similar frequencies of AMR gene families present in global influent and effluent samples. Multiple β-lactams such as GOB, IND, BJP, and EC beta-lactamases can only be found in effluent samples, further supporting results by Waśko et al. However, aph(3”) genes were only detected in influent samples and sulfonamide resistance genes had similar frequencies in both influent and effluent samples, which contradicts the findings of Raza et al who found significantly more sul1 and APH(3ʹʹ)-lb genes in effluent samples from Seoul, South Korea [34]. South Korean samples collected in this study are from the city of Gwangju, and the difference in geographical location and cities can be attributed to the difference in ARG frequencies as highlighted by J. Bengtsson-Palme et al in their 2023 review of monitoring AMR in the environment where they mention monitoring AMR in waterways such as sewage arguably reflects the local AMR situation [35].
3.3. Many ARGs confer resistance to ‘access’ and ‘watch’ antibiotics in global WWTP influent and effluent
The World Health Organization (WHO) classifies antibiotics as ‘access’, ‘watch’, and ‘reserve’ as part of the AWaRe classification [36]. ‘Access’ group antibiotics work against a large range of common pathogens and have low resistance potentials, while ‘watch’ antibiotics have higher resistance potentials than ‘access’ antibiotics. ‘Reserve’ antibiotics are reserved to treat multi-drug-resistant pathogens [37].
Drug classes to which ARGs in global WWTP influent and effluent samples confer resistance to were combined and their frequencies were plotted in a stacked bar graph (Figure 7). The drug classes were then categorized based on the AWaRe classification (Table 3), and a pie-chart of the distribution of the AWaRe classification was created (Figure 8). Drug classes not present in the AWaRe classification were classified as ‘NA’.
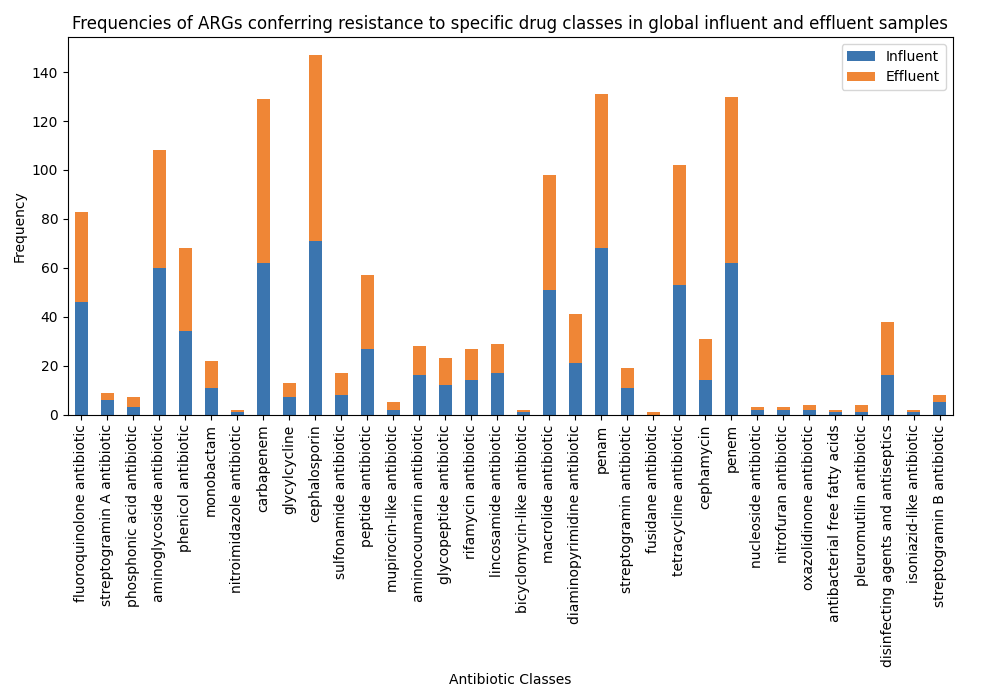
Drug Class | AWaRe Classification |
Fluoroquinolone antibiotic | Watch |
Streptogramin antibiotic | Reserve |
Phosphonic acid antibiotic | Reserve |
Aminoglycoside antibiotic | Access |
Phenicol antibiotic | Access |
Monobactam antibiotic | Reserve |
Nitroimidazole antibiotic | NA |
Carbapenem | Watch |
Glycylcycline | Reserve |
Cephalosporin | Access |
Sulfonamide (with trimethoprim) | Access |
Peptide antibiotic | Watch |
Mupirocin-like antibiotic | NA |
Aminocoumarin antibiotic | NA |
Glycopeptide antibiotic | Reserve |
Rifamycin antibiotic | Watch |
Lincosamide antibiotic | Access |
Bicyclomycin-like antibiotic | NA |
Macrolide antibiotic | Watch |
Diaminopyrimidine antibiotic | NA |
Penem | NA |
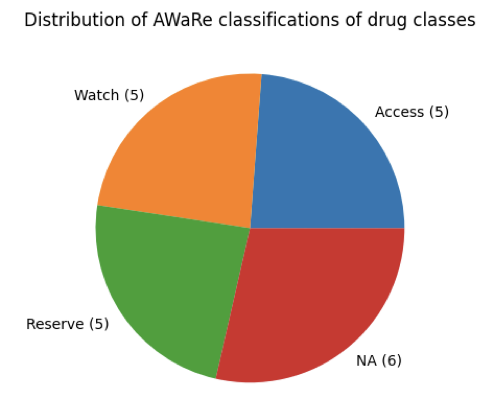
Equal numbers of drug classes were categorized as ‘access’, ‘watch’, and ‘reserve’ (5 each). ‘Access’ and ‘watch’ antibiotics are critical for the functioning of public health systems. With ARGs conferring resistance to ‘access’ and ‘watch’ antibiotics being present just as much as ARGs conferring resistance to ‘reserve’ antibiotics, the risk of resistance against ‘access’ and ‘watch’ antibiotics increases. The wide-spread presence of ARGs conferring resistance to crucial antimicrobials in WWTP influent and effluent highlights the need for increased antimicrobial stewardship, surveillance, and methods to remove ARGs and resistant pathogenic microbes from WWTP influent.
3.4. Tackling AMR in WWTPs
Multiple methods have been proposed to remove resistant microbes and ARGs from WWTP influent. For example, Pant et al. described adsorption techniques whereby ARGs bind to the surfaces of nanoparticles in their review of removal of antimicrobial resistance from secondary treated wastewater [38]. Grimes et al also highlighted using algae-mediated bioremediation to remove plasmids (pEX18Tc) carrying a tetracycline resistant gene [39]. These novel techniques combined with increased surveillance efforts for AMR in the environment can help tackle AMR in WWTPs.
Conclusion
Distributions and frequencies of resistant pathogenic microbes and ARGs from global wastewater treatment plant influent and effluent samples were compared using a culture-independent metagenomics approach. It was found that there were similar distributions and frequencies of resistant pathogens and ARGs in global influent and effluent. ARGs conferring resistance to clinically crucial drug classes were also found in high frequencies in global influent and effluent samples.
References
[1] THE REVIEW ON ANTIMICROBIAL RESISTANCE and J. O’NEILL, “TACKLING DRUG-RESISTANT INFECTIONS GLOBALLY: FINAL REPORT AND RECOMMENDATIONS,” Apr. 2016. [Online]. Available: https://amr-review.org/sites/default/files/160518_Final%20paper_with%20cover.pdf
[2] “To reduce superbugs, world must cut down pollution,” UN Environment. https://www.unep.org/news-and-stories/press-release/reduce-superbugs-world-must-cut-down-pollution
[3] A. K. Rad et al., “An overview of antibiotic resistance and abiotic stresses affecting antimicrobial resistance in agricultural soils,” International Journal of Environmental Research and Public Health/International Journal of Environmental Research and Public Health, vol. 19, no. 8, p. 4666, Apr. 2022, doi: 10.3390/ijerph19084666
[4] “AMRmageddon: the Material Risks of AMR to Animal Health and the Livestock Sector | FAIRR.” https://www.fairr.org/news-events/insights/amr-material-risks-animal-health
[5] World Health Organization: WHO, “Antimicrobial resistance,” Nov. 21, 2023. https://www.who.int/news-room/fact-sheets/detail/antimicrobial-resistance#:~:text=AMR%20is%20a%20natural%20process,through%20genetic%20changes%20in%20pathogens
[6] Partridge, S. R., Kwong, S. M., Firth, N., & Jensen, S. O. (2018). Mobile Genetic Elements Associated with Antimicrobial Resistance. Clinical microbiology reviews, 31(4), e00088-17. https://doi.org/10.1128/CMR.00088-17
[7] Dmitrijeva, M., Tackmann, J., Matias Rodrigues, J.F. et al. A global survey of prokaryotic genomes reveals the eco-evolutionary pressures driving horizontal gene transfer. Nat Ecol Evol 8, 986–998 (2024). https://doi.org/10.1038/s41559-024-02357-0
[8] “Antimicrobial resistance: the Complexity of Transmission – JPIAMR.” https://www.jpiamr.eu/antimicrobial-resistance-the-complexity-of-transmission/
[9] Amarasiri, M., Sano, D., & Suzuki, S. (2019). Understanding human health risks caused by antibiotic resistant bacteria (ARB) and antibiotic resistance genes (ARG) in water environments: Current knowledge and questions to be answered. Critical Reviews in Environmental Science and Technology, 50(19), 2016–2059. https://doi.org/10.1080/10643389.2019.1692611
[10] A. K. Singh, R. Kaur, S. Verma, and S. Singh, “Antimicrobials and antibiotic resistance genes in water bodies: Pollution, risk, and control,” Frontiers in Environmental Science, vol. 10, Apr. 2022, doi: 10.3389/fenvs.2022.830861.
[11] Li, J., Cheng, W., Xu, L., Strong, P. J., & Chen, H. (2015). Antibiotic-resistant genes and antibiotic-resistant bacteria in the effluent of urban residential areas, hospitals, and a municipal wastewater treatment plant system. Environmental Science and Pollution Research, 22(6), 4587–4596. doi:10.1007/s11356-014-3665-2
[12] Pruden, A., Pei, R., Storteboom, H., & Carlson, K. H. (2006). Antibiotic resistance genes as emerging contaminants: Studies in Northern Colorado. Environmental Science & Technology, 40(23), 7445–7450. doi:10.1021/es060413l
[13] Rodriguez-Mozaz, S., Chamorro, S., Marti, E., Huerta, B., Gros, M., Sànchez-Melsió, A., … Balcázar, J. L. (2015). Occurrence of antibiotics and antibiotic resistance genes in hospital and urban wastewaters and their impact on the receiving river. Water Research, 69, 234–242. doi:10.1016/j.watres.2014.11.021
[14] A. Navarro et al., “Study of antibiotic resistance in freshwater ecosystems with low anthropogenic impact,” Science of the Total Environment, vol. 857, p. 159378, Jan. 2023, doi: 10.1016/j.scitotenv.2022.159378
[15] Singh R., Singh A.P., Kumar S., Giri B.S., Kim K.-H. Antibiotic Resistance in Major Rivers in the World: A Systematic Review on Occurrence, Emergence, and Management Strategies. J. Clean. Prod. 2019;234:1484–1505. doi: 10.1016/j.jclepro.2019.06.243
[16] Pruden A., Vikesland P.J., Davis B.C., de Roda Husman A.M. Seizing the Moment: Now Is the Time for Integrated Global Surveillance of Antimicrobial Resistance in Wastewater Environments. Curr. Opin. Microbiol. 2021;64:91–99. doi: 10.1016/j.mib.2021.09.013.
[17] Honda, R., Matsuura, N., Sorn, S. et al. Transition of antimicrobial resistome in wastewater treatment plants: impact of process configuration, geographical location and season. npj Clean Water 6, 46 (2023). https://doi.org/10.1038/s41545-023-00261-x
[18] Alexander, J., Hembach, N. & Schwartz, T. Evaluation of antibiotic resistance dissemination by wastewater treatment plant effluents with different catchment areas in Germany. Sci Rep 10, 8952 (2020). https://doi.org/10.1038/s41598-020-65635-4
[19] Lira, F., Vaz-Moreira, I., Tamames, J. et al. Metagenomic analysis of an urban resistome before and after wastewater treatment. Sci Rep 10, 8174 (2020). https://doi.org/10.1038/s41598-020-65031-y
[20] Lee, J., Ju, F., Beck, K. et al. Differential effects of wastewater treatment plant effluents on the antibiotic resistomes of diverse river habitats. ISME J 17, 1993–2002 (2023). https://doi.org/10.1038/s41396-023-01506-w
[21] A. Gholizadeh, M. Khiadani, M. Foroughi, H. A. Siuki, and H. Mehrfar, “Wastewater treatment plants: The missing link in global One-Health surveillance and management of antibiotic resistance,” Journal of Infection and Public Health, vol. 16, pp. 217–224, Dec. 2023, doi: 10.1016/j.jiph.2023.09.017.
[22] L. Zhang et al., “Advances in metagenomics and its application in environmental microorganisms,” Frontiers in Microbiology, vol. 12, Dec. 2021, doi: 10.3389/fmicb.2021.766364.
[23] Nam, N. N., Do, H. D. K., Loan Trinh, K. T., & Lee, N. Y. (2023). Metagenomics: An Effective Approach for Exploring Microbial Diversity and Functions. Foods (Basel, Switzerland), 12(11), 2140. https://doi.org/10.3390/foods12112140
[24] J. D. Forbes, N. C. Knox, J. Ronholm, F. Pagotto, and A. Reimer, “Metagenomics: the next Culture-Independent game changer,” Frontiers in Microbiology, vol. 8, Jul. 2017, doi: 10.3389/fmicb.2017.01069.
[25] Kalantar, Katrina L., et al. “IDseq—An open source cloud-based pipeline and analysis service for metagenomic pathogen detection and monitoring.” Gigascience 9.10 (2020)
[26] B. W. N. Grehs, M. a. O. Linton, B. Clasen, A. De Oliveira Silveira, and E. Carissimi, “Antibiotic resistance in wastewater treatment plants: understanding the problem and future perspectives,” Archives of Microbiology, vol. 203, no. 3, pp. 1009–1020, Oct. 2020, doi: 10.1007/s00203-020-02093-6.
[27] Josephine Burgin, Alisha Ahamed, Carla Cummins, Rajkumar Devraj, Khadim Gueye, Dipayan Gupta, Vikas Gupta, Muhammad Haseeb, Maira Ihsan, Eugene Ivanov, Suran Jayathilaka, Vishnukumar Balavenkataraman Kadhirvelu, Manish Kumar, Ankur Lathi, Rasko Leinonen, Milena Mansurova, Jasmine McKinnon, Colman O’Cathail, Joana Paupério, Stéphane Pesant, Nadim Rahman, Gabriele Rinck, Sandeep Selvakumar, Swati Suman, Senthilnathan Vijayaraja, Zahra Waheed, Peter Woollard, David Yuan, Ahmad Zyoud, Tony Burdett, Guy Cochrane, The European Nucleotide Archive in 2022, Nucleic Acids Research, Volume 51, Issue D1, 6 January 2023, Pages D121–D125, https://doi.org/10.1093/nar/gkac1051
[28] “Home – SRA – NCBI.” https://www.ncbi.nlm.nih.gov/sra
[29] CZ ID pipeline overviews. (n.d.). CZ ID Help Center. https://chanzuckerberg.zendesk.com/hc/en-us/articles/13756558532884-CZ-ID-Pipeline-Overviews
[30] Alcock, B. P., Huynh, W., Chalil, R., Smith, K. W., Raphenya, A. R., Wlodarski, M. A., Edalatmand, A., Petkau, A., Syed, S. A., Tsang, K. K., Baker, S. J. C., Dave, M., McCarthy, M. C., Mukiri, K. M., Nasir, J. A., Golbon, B., Imtiaz, H., Jiang, X., Kaur, K., Kwong, M., … McArthur, A. G. (2023). CARD 2023: expanded curation, support for machine learning, and resistome prediction at the Comprehensive Antibiotic Resistance Database. Nucleic acids research, 51(D1), D690–D699. https://doi.org/10.1093/nar/gkac920
[31] Pine, P.S., Munro, S.A., Parsons, J.R. et al. Evaluation of the External RNA Controls Consortium (ERCC) reference material using a modified Latin square design. BMC Biotechnol 16, 54 (2016). https://doi.org/10.1186/s12896-016-0281-x
[32] Pauli Virtanen, Ralf Gommers, Travis E. Oliphant, Matt Haberland, Tyler Reddy, David Cournapeau, Evgeni Burovski, Pearu Peterson, Warren Weckesser, Jonathan Bright, Stéfan J. van der Walt, Matthew Brett, Joshua Wilson, K. Jarrod Millman, Nikolay Mayorov, Andrew R. J. Nelson, Eric Jones, Robert Kern, Eric Larson, CJ Carey, İlhan Polat, Yu Feng, Eric W. Moore, Jake VanderPlas, Denis Laxalde, Josef Perktold, Robert Cimrman, Ian Henriksen, E.A. Quintero, Charles R Harris, Anne M. Archibald, Antônio H. Ribeiro, Fabian Pedregosa, Paul van Mulbregt, and SciPy 1.0 Contributors. (2020) SciPy 1.0: Fundamental Algorithms for Scientific Computing in Python. Nature Methods, 17(3), 261-272
[33] I. Waśko, A. Kozińska, E. Kotlarska, and A. Baraniak, “Clinically relevant Β-Lactam resistance genes in wastewater treatment plants,” International Journal of Environmental Research and Public Health/International Journal of Environmental Research and Public Health, vol. 19, no. 21, p. 13829, Oct. 2022, doi: 10.3390/ijerph192113829.
[34] S. Raza, H. Shin, H.-G. Hur, and T. Unno, “Higher abundance of core antimicrobial resistant genes in effluent from wastewater treatment plants,” Water Research, vol. 208, p. 117882, Jan. 2022, doi: 10.1016/j.watres.2021.117882.
[35] J. Bengtsson-Palme et al., “Towards monitoring of antimicrobial resistance in the environment: For what reasons, how to implement it, and what are the data needs?,” Environment International, vol. 178, p. 108089, Aug. 2023, doi: 10.1016/j.envint.2023.108089
[36] Essential Medicines (EML), “2021 AWaRe classification,” Sep. 30, 2021. https://www.who.int/publications/i/item/2021-aware-classification
[37] World Health Organization: WHO, “WHO releases the 2019 AWARE Classification Antibiotics,” WHO, Oct. 01, 2019. [Online]. Available: https://www.who.int/news/item/01-10-2019-who-releases-the-2019-aware-classification-antibiotics
[38] A. Pant, M. Shahadat, S. W. Ali, and S. Z. Ahammad, “Removal of antimicrobial resistance from secondary treated wastewater – A review,” Journal of Hazardous Materials Advances, vol. 8, p. 100189, Nov. 2022, doi: 10.1016/j.hazadv.2022.100189
[39] K. L. Grimes, L. J. Dunphy, G. L. Kolling, J. A. Papin, and L. M. Colosi, “Algae-mediated treatment offers apparent removal of a model antibiotic resistance gene,” Algal Research, vol. 60, p. 102540, Dec. 2021, doi: 10.1016/j.algal.2021.102540.
About the author
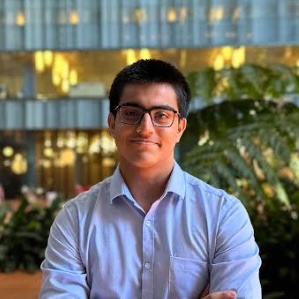
Vedanth Ramji
Vedanth is a high school senior at APL Global School in Chennai, India and a long-term student researcher at the Big Data Biology Lab. He is interested in environmental metagenomics, especially understanding antimicrobial resistance in our surroundings.