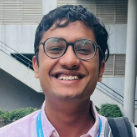
Author: Akarsh Gupta
Mentor: Dr. Hong Pan
National Institute of Technology Karnataka
Abstract
In the realm of neuroscience, brain mapping emerges as a pivotal method for comprehending the human brain’s structure and function. Utilizing Functional Magnetic Resonance Imaging (fMRI), researchers gain insights into real-time brain activity, offering unparalleled understanding of cognitive processes. The non-invasive nature and superior spatial resolution of fMRI distinguish it from other imaging modalities like PET scans and EEGs. However, interpreting fMRI data demands sophisticated analysis techniques, a challenge addressed by Statistical Parametric Mapping (SPM). SPM serves as a robust software tool for preprocessing, statistical analysis, and visualization of neuroimaging data, including fMRI studies. In this paper, we focus on group-level analysis of fMRI data from a cohort subjected to the Eriksen Flanker task, utilizing SPM12 for analysis. The paper provides an in-depth tutorial on SPM12, covering preprocessing steps and group-level analysis procedures. By integrating fMRI with SPM analysis, researchers gain unprecedented insights into neural activity patterns underlying perception, cognition, and behavior. This synthesis of cutting-edge technology and analytical tools offers a gateway to understanding the complexities of the human mind, with implications for advancing neuroscience research and clinical applications.
Keywords
Voxel – A three-dimensional pixel, representing a volume element in a digital image or dataset
Timing Files – A data file that contains precise information, often in the form of timestamps or time intervals, used to synchronize experimental events with neuroimaging data during analysis
Run – Refers to a single session of data acquisition during neuroimaging experiment, typically capturing brain activity over a specific duration or set of experimental conditions.
T-distribution – A probability distribution that arises from estimating the mean of a normally distributed population when the sample size is small or the population standard deviation is unknown.
SPM12 – A sophisticated software package used for the analysis of neuroimaging data, offering a comprehensive suite of tools for preprocessing, statistical analysis, and visualization.
Introduction
In the realm of neuroscience, one of the most captivating frontiers is the exploration of the human brain. From deciphering its intricate architecture to understanding its complex functionalities, researchers have been long engaged in unraveling the mysteries of this organ. At the heart of this pursuit lies brain mapping, a multidisciplinary method that harmonizes diverse imaging techniques to visualize and understand both the structure and function of the brain. (Li et al., 2014)
Functional Magnetic Resonance Imaging or fMRI enables researchers to observe brain activity in real-life, offering unprecedented insights into the neural processes underlying perception, cognition, emotion, and behaviour.The biggest advantage of using fMRI rather than a PET Scan or an EEG, is that it is essentially non-invasive and returns imaging of good spatial resolution.(Pan et al., 2011) By detecting changes in blood flow and oxygenation levels associated with neuronal activity, fMRI provides a non-invasive window into the brain’s functional architecture. However, making any sense of extracting any useful information from this fMRI data requires advanced analysis techniques adept at distinguishing genuine neural signals from background noise, while pinpointing activity patterns linked to particular cognitive tasks or experimental parameters.
Statistical Parametric Mapping (SPM) stands as a robust software tool extensively employed in the analysis of neuroimaging data, encompassing fMRI studies. SPM offers a comprehensive suite of tools for preprocessing, statistical analysis for groups of subjects, and visualization of brain images. Its adaptable framework enables the modeling of intricate experimental setups, evaluation of observed effects significance, and creation of compelling visual representations to convey findings with precision.(Barone et al., 2018)
In this paper, we dive head-first into the vast realm of brain mapping, focusing specifically on the utilization of fMRI and SPM analysis. We are conducting group level analysis on a group of 20 subjects that were subjected to the Eriksen Flanker task.(Servant & Logan, 2019) We also provide an in-depth introduction to SPM12, guiding readers through the steps involved in analyzing the fMRI data that we have. Figure 1 gives us an accurate illustration of the Flanker paradigm.

By merging the advanced capabilities of fMRI technology with the analytical prowess of SPM, researchers gain unprecedented access to the inner workings of the human mind. This powerful combination enables us to explore the intricacies of neural activity, shedding light on the mechanisms behind perception, cognition, and behavior.
Metholodogy

fMRI Machine

Figure 3 depicts an fMRI machine that is used to assess how the brain is working. Hospitals extensively use these machines to help determine potential risk of surgeries or other invasive procedures. The scans from these machines help diagnose strokes, brain tumors, brain injuries, Alzheimer’s Disease, Epilepsy and so much more.
From a research standpoint, Researchers use these scans to help them understand which part of the brain is associated with which action which can be pivotal in studies that help them in the process of brain-mapping. An fMRI imaging scan takes advantage of activated neurons requiring more oxygen from red blood cells. This increase in activity leads to a change in blood flow. fMRI detects these changes. By indirectly measuring the alterations in blood flow and electrical activity, fMRI assesses brain activity.
The several benefits of using functional MRI scan are firstly, the nature of it being noninvasive, which means that it does not require surgery to obtain. Secondly, the versatility of fMRI scans allows researchers to assess both structure and function of the brain.
Dataset
The dataset comprises data collected from 20 healthy adults while they performed a slow event-related Eriksen Flanker Task. During the interval of each trial, The participants used one of the two buttons that were present on the response pad to indicate the direction of the central arrow in a sequence of 5 arrows. In the congruent trials, the flanking arrows point in the same direction as the central arrow, for example, (< < < < <), while in more demanding incongruent trials the flanking arrows pointed in the opposite direction, for example , ( < < > < <).
The subjects performed two 5-minutes blocks, each containing 12 congruent and 12 incongruent trials which were presented in a pseudorandom order.
Resulting data obtained is 146 contiguous echo planar imaging (EPI) whole – brain functional volumes during both the congruent as well as the incongruent task blocks. A high resolution T1-weighted anatomical image was also acquired using a magnetization prepared gradient echo sequence.
The data collected has 146 continuous images of the entire brain using a technique called Echo Planar Imaging(EPI), which is a MRI technique used to rapidly acquire images. This method allows us to capture brain activity while the subjects complete tasks where they match or mismatch stimuli. Additionally, detailed pictures of the brain’s structure were taken which had excellent contrast between different types of brain tissue.(Iturria-Medina et al., 2008)

Figure 4: (A) displays the anatomical image of subject-08, providing a detailed representation of the brain’s structure. (B) displays the functional image of subject-08. Capturing dynamic changes in brain activity during a specific task or resting state.
Preprocessing
After downloading the data, we have to clean the brain imaging data so that it can be later used for group analysis. An fMRI volume contains not only the signal that we are interested in changes in oxygenated blood – but also fluctuations that we are not interested in, such as head motion, random drifts, breathing, and heartbeats. We call these other fluctuations noise, since we want to separate them from the signal that we are interested in. This noise will be removed by the preprocessing methods that we shall employ (Ganzetti et al., 2018). Figure 5 shows comprehensive process of acquiring functional MRI images. This process was followed for our 20 subjects.


Realignment
The brain imagining data is a time-series one. Time-series data can be thought of as a deck of cards, where each volume is a different card. The first step that comes in preprocessing of this data is Realignment. Basically what realignment does is it will put all the cards in the same orientation and all the sides line up.
Slice-Timing Correction
Unlike other photographic data, in which the entire picture is taken in a single moment, an fMRI volume is acquired in slices. Each of these slices takes time to acquire – from tens to hundreds of milliseconds. There are 2 commonly used methods for creating volumes, which are:
1) sequential slice acquisition
2) interleaved slice acquisition
Coregistration
Although most people’s brains are similar – everyone has a hypothalamus and the cingulate gyrus for example – there are also differences in brain size and shape. Due to this difference, group analysis is not possible unless each voxel for each subject corresponds to the same part of the brain. This essentially means that for example, if we are studying a voxel in the visual cortex, for the group analysis we would want to make sure that the visual cortex for all the subjects are aligned.
This will be employed by Registering and Normalizing the data. This could be thought of as folding clothes to fit them inside of a suitcase, similarly each brain image needs to be reconstructed to have the same size, shape and dimensions. We warp our data to a template. A template serves as a standardized framework with predefined dimensions and coordinates, universally adopted by researchers for reporting their findings. Before we move onto seeing how Registering and Normalizing works, we must define what Affine Transformation is to better understand the end-to-end process of Coregistration.
Affine Transformations
Affine Transformations are what help warp our images to the template that we use. It is very similar to the rigid-body transformation which we have described above in Motion Correction, however it adds to it by adding two more dimensions, namely – Zooms and Shears. Translations and rotations are actions that can be done with everyday items such as pencils, whereas zoom and shear are more unusual – zooms either shrink or enlarge the image, while shears take the diagonally opposite corners of the image and stretch them away from each other.
Registration
Our goal is to warp the functional images to the template so that we can finally perform a group-level analysis across all our subjects. While it might appear logical to directly warp the functional images onto the template, this approach often proves ineffective in practice. This is because the low-resolution of the images makes it less probable for them to align accurately with the anatomical details of the template. Consequently, the anatomical image presents a superior option for this purpose.
While performing this step doesn’t get us any closer to the final goal, in turn warping the anatomical image can assist with bringing the functional images into standardized space. Since our anatomical image is already normalized to a template and recorded what transformations are done, we can apply the same transformations to the functional images.
When aligning functional and anatomical images, it’s crucial to ensure they are roughly in the same location. The outlines of the images must be aligned accordingly if they aren’t. The initial step sets the foundation for accurate registration.
A key advantage lies in the distinct contrast weightings of anatomical and functional images. Dark areas in the anatomical image, like cerebrospinal fluid, appear bright in the functional image, and vice versa. Leveraging the mutual information, the registration algorithm strategically manipulates the images to explore various overlays. It seeks to match bright voxels in one image with dark voxels in the other, and vice versa, iteratively testing until it finds the optimal alignment. The iterative process is driven by a cost function, aiming to maximize alignment quality.
Segmentation
The brain consists primarily of two tissue types: Grey Matter, which contains dense concentrations of unmyelinated neurons, and White Matter, which contains dense concentrations of myelinated neurons. Surrounding the brain is Cerebrospinal Fluid(CSF), with significant amounts found within internal spaces known as ventricles.
It is essential to map each voxel to their corresponding tissue type, for normalizing the anatomical image, while aligning it to the standardized template. SPM utilizes six tissue priors, each representing an estimation of tissue distribution in standardized space. (Ashburner & Friston, 2005)
Normalization
Normalization marks the final step for preprocessing of the data. It is essential to finally bring our data to form on which we can perform group-level analysis. After the anatomical image has been segmented, we can use those segmentations to normalize our images.
While Normalizing using the SPM GUI, we have used the default values for voxel resolution which is 2x2x2. The reason this value is taken is because it creates higher-resolution images. One down side of this is the larger space required to store these images.(3x3x3 can be used but will avail smaller files with lower resolution)
Smoothing
fMRI data contains a lot of noise, and this noise usually outweighs the signal itself. To combat this noise, we use smoothing to reduce this noise. Smoothing entails replacing each voxel with a weighted average of that voxel’s neighbors. Even though we are making the image blurrier, the noise reduction proves to make a significant change in the later stage when we are performing group analysis.
We have now performed all the necessary steps required for preprocessing and can move onto the first level analysis
Statistical Analysis
Creating the Ideal Time-Series
Before we fit a model to our fMRI data, we know that our data is a time-series one. So we need the pertaining fitted time-series so that we can use the estimated beta weights in our group-level analysis.
Within our dataset, under each subject’s directory there is a “func” directory, there is a file named events.tsv. This file contains information that is required to create our timing files which are the name of the condition(i.e. Incongruent or congruent), the instance the condition took place(in seconds) relative to the start of the scan and lastly the duration of each trial. Once this information was extracted and formatted in a way that the SPM software can utilize it, we further created a timing file for each condition and then split that file according to which run the condition was in. This resulted in 4 timing files that were essentially:
- Timings for the Incongruent trials that occurred during the first run (which we will call incongruent_run1.txt);
- Timings for the Incongruent trials that occurred during the second run (incongruent_run2.txt);
- Timings for the Congruent trials that occurred during the first run (congruent_run1.txt);
- Timings for the Congruent trials that occurred during the second run (congruent_run2.txt).
All the timing files adhere to a consistent format, comprising of two columns namely, Onset time, in seconds, relative to the start of the scan and Duration of the trial, in seconds,
Figure 7 shows us the original.tsv file and how it is structured and then we use a script to allow us to transform this data into the required form that we need to help us fit a model in the SPM software. Figure 7 (B) has two columns like we discussed before, the leftmost one being the Onset time, in seconds and the rightmost one being the duration of the trial, in seconds.

Running The First-level Analysis
Specifying the Model
Having created the timing files in the above subsection, we can now finally use them in conjunction with the imaging data to create Statistical Parametric Maps. These maps essentially indicate the strength of the correlation between the ideal time-series and the time-series data that we got during the course of the experiment. The beta weights obtained for the multiple regressor in the model in turn are converted into a t-distribution.
To keep things organized, we have created a new directory named 1stLevel in each subject directory. Now, navigating to “Specify 1st-Level” on the SPM GUI, we start by entering the data.
For the directory, we pick the 1stLevel for the current subject, i.e. Sub-08. Next comes filling in the Timing patterns, for this we are using a value of 2 seconds for the Interscan Interval. Now we want to have 2 sessions because in our dataset, we have two runs of each subject. So we fill in the data with the 2 sessions. Followed by the setting of data, we also have to set the two conditions, which are Incongruent and Congruent in this case. Lastly, we need the onset time for each occurrence of the incongruent as well as congruent condition. Since in this experiment the duration of each trial lasted for 2 seconds, the value of the duration field is set to 2 seconds. Once this was done, we ran the following parameters.
The resulting GLM (General Linear Model) is generated and looks like the figure shown below.

Estimating the Model
Now that we have the General Linear Model or GLM, the next step is to estimate the beta weights for each condition. We navigate to the Estimate option on the SPM GUI, and select the SPM.mat file that was created in the 1stLevel directory in Sub-08’s directory and run it. Before being able to result, we need to create a contrast first. Essentially what we are doing is that, if we have a beta weight for the incongruent condition and a beta weight for the congruent condition, we can take the difference of them to calculate a contrast estimate at each voxel in the brain. After doing this task for every single voxel we will basically create a contrast map.
To create these contrasts, we navigate to the Results button of the SPM GUI, selecting the SPM.mat file that was generated after estimating the model. We will be greeted by a new window, with an empty design matrix on the right side of the panel. The next step is to define the new contrast with a name, in this case Inc-Con and a contrast vector of [0.5 -0.5 0.5 -0.5] and then we create this contrast.
In contrast, the reason we are using values of 0.5 and -0.5, instead of 1 and -1, it is because of accounting for the number of runs in the study, which is 2. To make the results comparable to other subjects or even other studies that may even try different amounts of runs. Hence, the contrast weights of 1 and -1 are divided by the number of runs that we have taken, i.e. 1 / 2 = 0.5 and -1 / 2 = -0.5.
Now let us use this contrast, once double-clicked, we had to choose a few more options, which were:
- Apply masking: We set this to “none”, as we wanted to examine all the voxels in the brain.
- p value: Again set this to none and set the uncorrected p-value to 0.01. This essentially tests each voxel at a p-threshold of 0.01.
- Extent threshold (voxels): We have set this value to 10, which means the result will only show clusters of 10 or more contiguous voxels. We are setting it at 10, to avoid the specks of voxels that may show up due to them being in noisy regions.
After filling this information, we are finally displayed with the first-level analysis as seen in Figure 9. The result is displayed on a glass brain. The dark spots in the standardized space are the clusters of voxels that passed our statistical thresholds. A plus point is that we can see all the locations as well as the statistical significance of each cluster. Set Level informs us on the probability of seeing the current number of voxels. The Cluster-level column shows the significance for each cluster using different correction methods. The Peak-Level column informs us the t- and z-statistics of the peak voxel within each cluster, with the main clusters marked in bold and any sub-clusters listed below the main cluster marked in light font. On the right most side, we have the MNI coordinates of the peaks of each cluster and sub-cluster listed.
Figure 9 shows us a comprehensive view about the different regions of interest within the statistics table such as set level, cluster-level, peak-level and the MNI coordinates. From the glass brain, we can see that one of the groups of voxels that are statistically significant is in the area containing the Dorsal Medial Prefrontal Cortex. (Maruyama et al., 2018)

This entire process was repeated for the remaining 19 subjects. Now we can move on to generalizing the results in the group analysis.
Group Analysis
Specifying the Model
Now that we have done our first level analysis on all the subjects. We can now move on to see how the results fare in the entire population and for us to draw our inferences from the Eriksen Flanker Task. To test this, we shall run a 2nd Level analysis, which essentially calculates the standard error and the mean for a contrast estimate, and then test whether the average estimate is statistically significant or not. This method conducts group-level analysis using a summary statistic approach that disregards variability in parameter estimates. Instead, it employs a t-test on the mean parameter estimates obtained from each subject.
Like we did for the first level analysis, where we created a directory for the result, we do the same here but created one directory in the root directory and named it 2ndLevel_Inc-Con. We then proceeded to the SPM GUI and clicked on Specify 2nd-Level. We were required to just fill in the location for the output to be placed and that would be the new directory we created and the scans that we needed to conduct the test on, which were the 1st Level analysis outputs of each subject. After everything was filled, we ran the test.
Estimating the Model
After specification, we needed to estimate our model. The procedure is exactly the same for when we performed it for the 1st Level estimate, however we use the SPM.mat file created. Once this was done, we were ready to check the results. We had to create a new contrast for the 2nd Level Analysis. There is only one regressor, hence we only have to define one weight in the weight vector,namely 1 (Han & Park, 2018). We named this contrast Inc-Con. Once we completed creating the contrast, we were prompted to fill in the following:
- Apply masking -> None
- p value adjustment to control -> None
- Threshold (T or p value) -> 0.01
- Extent Threshold (voxels) -> 20
The process of how 2nd Level Analysis is shown in Figure 10, how each 1st Level Analysis result is taken into account to generalize the showings. Again in the statistics table that is presented after the 2nd Level Analysis shows that the Dorsal Medial Prefrontal Cortex has a significant cluster shown by the red circle over the statistics table. (Barone et al., 2018)

contrast estimate, and the finally test whether the average estimate is statistically significant. We can see the areas that are significant, glowing in the glass brain. The red circle encircles the
location of the Dorsal Medial Prefrontal Cortex, which is responsible with decision making.
For better visualization of the same result, however let us take a look at using MRIcroGL. It offers advantages in terms of real-time visualizations, three-dimensional rendering, and customizable display options. Additionally, MRIcroGL provides a user-friendly interface and intuitive navigation tools, making it particularly useful for researchers who prioritize interactive exploration and detailed inspection of brain structures and functional activations.

Conclusions
To summarize, brain mapping using various softwares such as SPM, can help us understand and detect how different parts of the brain are activated based on the activity performed. Like we see in the Eriksen Flanker Task, a psychological experiment that assesses selective attention and inhibitory function, subjects had to really focus on whether to press ‘<’ or ‘>’, in response to the Incongruent or Congruent scenario. When we performed our group level analysis, the dorsal medial prefrontal cortex, which is responsible for decision-making. It is observed to be more active in response to processing incongruent stimuli than congruent stimuli. This shows that the incongruent task requires more activity from certain parts of the brain that it would in the congruent task.
Just like the Eriksen Flanker Task, many more such tests can be run on the brain and recording the various active parts of the brain. Then using brain mapping, we can detect various Neurological conditions such as depression and anxiety. Brain mapping can also be used to detect Gliomas, the most common primary malignant brain tumor in adults which account for 80% of total cases. Which led to brain mapping also being used to measure the effectiveness of treatment using biomarkers, which are physical cues that are personalized to each patient’s brain.
On the other side of diseases, brain mapping has also been useful in understanding the brain. It can help us understand the brain’s connectivity and operations, and how humans learn. It can also help us develop visual insights into certain conditions, such as addiction or brain disorders. (Tavares et al., 2020)
As technology continues to evolve and interdisciplinary collaboration expands, the potential for brain mapping to unravel the mysteries of the mind and enhance human well-being is promising. With further research and refinement, brain mapping holds the key to unlocking the full potential of the most enigmatic organ in the human body.
References
Ashburner, J., & Friston, K. J. (2005). Unified segmentation. NeuroImage, 26(3), 839–851. https://doi.org/10.1016/j.neuroimage.2005.02.018
Barone, F., Alberio, N., Iacopino, D. G., Giammalva, G. R., D’Arrigo, C., Tagnese, W., Graziano, F., Cicero, S., & Maugeri, R. (2018). Brain Mapping as Helpful Tool in Brain Glioma Surgical Treatment—Toward the “Perfect Surgery”? Brain Sciences, 8(11), 192. https://doi.org/10.3390/brainsci8110192
Ganzetti, M., Liu, Q., Mantini, D., & Alzheimer’s Disease Neuroimaging Initiative. (2018). A Spatial Registration Toolbox for Structural MR Imaging of the Aging Brain. Neuroinformatics, 16(2), 167–179. https://doi.org/10.1007/s12021-018-9355-3
Han, H., & Park, J. (2018). Using SPM 12’s Second-Level Bayesian Inference Procedure for fMRI Analysis: Practical Guidelines for End Users. Frontiers in Neuroinformatics, 12. https://doi.org/10.3389/fninf.2018.00001
Huettel, S. A., Song, A. W., McCarthy, G., Huettel, S. A., Song, A. W., & McCarthy, G. (2014). Functional Magnetic Resonance Imaging (Third Edition, Third Edition). Oxford University Press.
Iturria-Medina, Y., Sotero, R. C., Canales-Rodríguez, E. J., Alemán-Gómez, Y., & Melie-García, L. (2008). Studying the human brain anatomical network via diffusion-weighted MRI and Graph Theory. NeuroImage, 40(3), 1064–1076. https://doi.org/10.1016/j.neuroimage.2007.10.060
Jiang, D., Zongyu, L., & Sun, G. (2021). The Effect of Yoga Meditation Practice on Young Adults’ Inhibitory Control: An fNIRS Study. Frontiers in Human Neuroscience, 15. https://doi.org/10.3389/fnhum.2021.725233
Li, Y., Yu, Z. L., Bi, N., Xu, Y., Gu, Z., & Amari, S. (2014). Sparse Representation for Brain Signal Processing: A tutorial on methods and applications. IEEE Signal Processing Magazine, 31(3), 96–106. https://doi.org/10.1109/MSP.2013.2296790
Mandal, P. K., Jindal, K., Roy, S., Arora, Y., Sharma, S., Joon, S., Goel, A., Ahasan, Z., Maroon, J. C., Singh, K., Sandal, K., Tripathi, M., Sharma, P., Samkaria, A., Gaur, S., & Shandilya, S. (2023). SWADESH: A multimodal multi-disease brain imaging and neuropsychological database and data analytics platform. Frontiers in Neurology, 14. https://doi.org/10.3389/fneur.2023.1258116
Maruyama, S., Muroi, K., & Hosokai, Y. (2018). Investigation of fMRI Analysis Method to Visualize the Difference in the Brain Activation Timing. Academic Radiology, 25(10), 1314–1317. https://doi.org/10.1016/j.acra.2018.01.026
Pan, H., Epstein, J., Silbersweig, D. A., & Stern, E. (2011). New and emerging imaging techniques for mapping brain circuitry. Brain Research Reviews, 67(1), 226–251. https://doi.org/10.1016/j.brainresrev.2011.02.004
Sarikahya, M. (2019). Age-related Changes in Conflict-related Activity in the Superior Frontal Gyrus: Implications for Cognitive Control. 7, 103–124.
Servant, M., & Logan, G. D. (2019). Dynamics of attentional focusing in the Eriksen flanker task. Attention, Perception, & Psychophysics, 81(8), 2710–2721. https://doi.org/10.3758/s13414-019-01796-3
Tavares, V., Prata, D., & Ferreira, H. A. (2020). Comparing SPM12 and CAT12 segmentation pipelines: A brain tissue volume-based age and Alzheimer’s disease study. Journal of Neuroscience Methods, 334, 108565. https://doi.org/10.1016/j.jneumeth.2019.108565
About the author
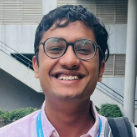
Akarsh Gupta
Akarsh is in his final year of studying at the National Institute of Technology, Karnataka Surathkal, India, where he is pursuing his major in Electrical and Electronics Engineering and his minor in Artifical Intelligence. Akarsh has a keen interest in conducting research in applied machine learning as well as Natural Language Processing. Akarsh is also an avid basketball player.