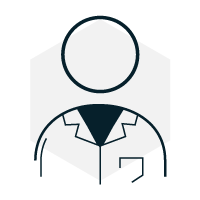
Author: Akshan Krithick
Mentor: Dr. Hong Pan
St.Joseph’s College of Engineering
Abstract
Changes in climate can affect ecosystems by influencing animal behavior and ranges. Birds are a particularly good indicator of environmental change as each species of bird has adapted or evolved to favor certain habitat types, food sources, and temperature ranges. In addition, the timing of certain events in their life cycles—such as migration and reproduction is driven by cues from the environment. Changing conditions can influence the distribution of both migratory and non-migratory birds as well as the timing of important life cycle events. Birds are easy to identify and count, and thus there is a wealth of scientific knowledge about their distribution and abundance. People have kept detailed records of bird observations for more than a century. There are many different species of birds living in a variety of habitats, including water birds, coastal birds, and land birds. If a change in behavior or range occurs across a range of bird types, it suggests that a common external factor might be the cause.
Birds are prone to the metabolic stress of long-distance movements due to unusual climatic events during their migration. After a large avian mortality event occurred in New Mexico in the fall of 2020, carcass studies indicated that starvation was the primary cause of death. Here we use a dataset consisting of 11 years of fall bird banding data from two locations, along with local drought indices, to determine what predicts avian health during the migration period. We use fat score data from over 15,000 individual birds to assess whether drought indices, age, diet, or residency influenced avian health using multiple logistic regression. Probability of positive fat scores decreased as drought severity increased for younger, insectivorous, migratory birds. Insectivores had a higher probability of receiving a fat score greater than zero relative to local drought conditions, which is important, since many North American insectivores are in steep decline. Migratory birds showed a greater response than year-round residents, and older birds showed a lower but significant response compared to hatch-year birds. Our results suggest that migratory insectivores in the southwestern United States may be less resilient to drought-related climate change.
Introduction
Understanding what makes species and ecosystems vulnerable to climate change has become integral to the field of conservation science. To date, climate change vulnerability assessments have predominantly focused on how species and ecosystems will respond to mean, long‐term changes in climate. Multiple extreme events are likely to act in synergistic ways to exacerbate risk of species’ extinction. For example, the co‐occurrence of drought and heat waves has greater impacts on bird abundance changes than if these events occur in isolation.. Birds migrate to move from areas of low or decreasing resources to areas of high or increasing resources. The two primary resources being sought are food and nesting locations. Another reason birds might migrate is due to the climatic conditions, that is migrating to a more favorable environment for the time being. Programs like North American Breeding Bird Survey [1] and Monitoring Avian Productivity and Survivorship (MAPS) program [2] ,help in bird banding during the breeding season. Over 1,175 MAPS stations have been established since the inception of the MAPS program in 1989 [3].The impetus for this research is how there is very less work available pertaining to bird health during fall migration [4]. This research is a reiteration of the one performed by Los Alamos National Laboratory under the name “Does Age, Residency, or Feeding Guild Coupled with a Drought Index Predict Avian Health during Fall Migration?” by Stanek, Jenna E., Brent E. Thompson, Sarah E. Milligan, Keegan A. Tranquillo, Stephen M. Fettig, and Charles D. Hathcock.
Birds are pushed to the edge of their physiological limits [5] during the migration period. Birds usually experience the largest annual morality at this period [6,7]. Many banding stations collect fall bird banding data, amount of fat in a migrating bird, have been used to assess fall migration timing in relation to weather [8,9,10,11]. In the fall of 2020, a cold weather event preceded by poor summer precipitation led to a large bird die-off in New Mexico [12]. The die-off was first attributed to just poor air quality from wildfire smoke on North America’s west coast and extreme fluctuating weather patterns. An upwards of one million birds might have died in this event [13]. Many of the dead birds were collected and submitted to the United States Geological Survey’s National Wildlife Health Center to determine the cause of death. The one consistent observation across most of the birds was that a high level of breakdown of muscle tissue in the breast meat, low or non-existent fat, and general emaciated conditions[12]. It can be stated that majority of these birds died from hypothermia. Avian migration is a complex and vital phenomenon that exposes birds to various environmental challenges during their journey. A significant factors affecting avian health during migration is the availability of food and water resources. In this research study, we investigate whether age, residency status, and feeding guild, when combined with a drought index, can be used as predictors of avian health during the fall migration period. The results provide valuable insights into the conservation efforts required to support avian populations during their migratory journeys.
Methods
The dataset consists of data pertaining to birds in the August to October time period collected from 2010 until 2020. The data includes birds from two different locations within north central New Mexico. These locations occur near the overlap of the Pacific and Central migratory bird flyways [14] and the migratory birds most likely come into the study area from both flyways. The birds were banded as per The North American Bird Banding Manual [15] . The identification and sexing criteria was based on the Identification Guide to North American Passerines [16] . Birds were grouped into either one of insectivore, granivore or omnivore on basis of their dieting habits as per life history information available from Cornell’s The Birds of North America Online [21] The birds were categorized as either breeding in the area or migrants. Our dataset consists of 15,244 individuals from 71 species, of which 44 species were considered to be migrants.
The Palmer Drought Severity Index (PDSI) [22] is a standardized index that spans from −10 (dry) to +10 (wet), and has been quite successful at quantifying long-term drought. PDSI value of 0.0 to −0.5 means the conditions are normal; −0.5 to −1.0 alludes to incipient drought; −1.0 to −2.0 corresponds to mild drought; −2.0 to −3.0 means moderate drought; −3.0 to −4.0 is severe drought and anything below -4.0 is classified as extreme drought as per The Climate Data Guide: Palmer Drought Severity Index (PDSI) [22].
Fat score is an estimate of the total tissue depth (fat + muscle). The categories within fat scores comprises of “none”, “trace”, “light”, “half”, “filled”, “bulging”, “greatly bulging”, and “very excessive”[2]. Fuel stores fluctuates seasonally[17] and that’s why fat score is a better metric of usage here than body mass[11]. The categories of fat scores here are set forth by the Institute for Bird Populations. Data from birds captured during the same year were excluded because we wanted to compare fat scores between the years. The “rare” species caught ≤ 3 times were removed from the 10-year dataset. The data is standardized for further analyses by grouping the subspecies into species category.
Multiple logistic regression models were employed to formulate the influence of age, residency, feeding guild coupled with a drought index. We grouped response variable of fat score into two values. One is “none”, which is equal to 0 and all the other categories are 1. The grouping is done in this manner to tackle zero inflation problems since nearly half of fat scores were categorized as “none”. If a migrant bird has a fat score greater than zero during fall migration, its in a better psychological condition. We use the R statistical software here and the libraries used are dplyr, tidyverse, ggplot2[18].
Model Selection
The Akaike information criterion (AIC) is an estimator of predictor error and thereby relative quality of statistical models for a given set of data. Given a collection of models for the data, AIC estimates the quality of each model, relative to each of the other models. Thus, AIC provides a means for model selection [19].
AIC is founded on information theory. When a statistical model is used to represent the process that generated the data, the representation will almost never be exact; so some information will be lost by using the model to represent the process. AIC estimates the relative amount of information lost by a given model: the less information a model loses, the higher the quality of that model. Also, more the number of predictor variables, it constitutes to a better model. In estimating the amount of information lost by a model, AIC deals with the trade-off between the goodness of fit of the model and the simplicity of the model. In other words, AIC deals with both the risk of overfitting and the risk of underfitting.
Model selection results of logistic regression models for estimating fat score in relation to Palmer Drought Severity Index (PDSI) from New Mexico region 2 climate division, diet classification (insectivore, granivore and omnivore), residency (migratory versus year-round resident) and age (hatch year versus after hatch year) with respect to the data collected from 2010 to 2020. Model selection based on Akaike’s Information Criterion (AIC), and difference in AIC between each model compared to the model with the lowest AIC (ΔAIC) is evaluated.
The candidate model set was based on combinations of variables contributing to avian health. correlated variables with a variance inflation factor >5 were not included in the same model or candidate set of models as per Regression Diagnostics: Identifying Influential Data and Sources of Collinearity [20].
Results
Our top model had no competing models that is; ΔAIC < 2. We found no significant evidence of neither over- dispersion nor under-dispersion. Our top model contained the variables PDSI, diet, residency, and age with interaction effects of PDSI for residency and diet.
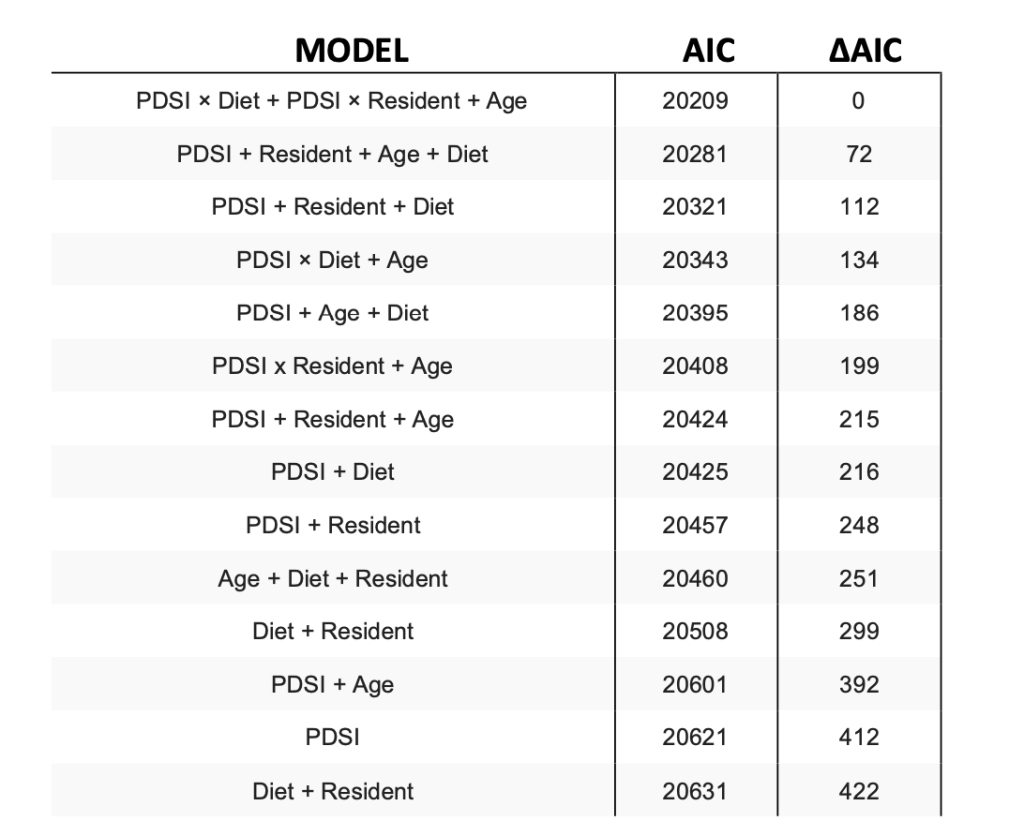
We can infer from our top model results that After Hatch-Year(AHY) birds had a higher probability of receiving a positive fat score when in comparison with Hatch-Year(HY) birds. Also, the insectivorous birds across both; year-round and migratory residency had higher probability of receiving a positive fat score when the conditions were wetter. In the case of omnivorous migratory birds and granivorous year-round birds, the conditions whether dry or wet did not affect the probability of them receiving a positive fat score across both ages(AHY or HY) but AHY birds had a higher probability of receiving a positive fat score compared to HY birds as inferred before. Granivorous migratory birds had a higher probability of receiving a positive fat score as the conditions became more wet. While considering the Year-round birds, omnivorous birds had a higher probability of receiving a positive fat score as the conditions were dry, as the conditions became wetter, the probability decreases.
Our findings emphasize the importance of considering age, residency status, feeding guild, and drought conditions when assessing avian health during fall migration. Conservation efforts should be tailored to address the specific needs of different age groups, resident vs. migratory populations, and feeding guilds to ensure the well-being and survival of migratory birds. In conclusion, age, residency status, feeding guild, and the drought index are important predictors of avian health during fall migration. Understanding these interrelationships provides valuable insights for conservation strategies, ensuring the preservation of migratory bird populations and maintaining ecosystem health.
References
- Ziolkowski, D., Jr.; Pardieck, K.; Sauer, J.R. On the road again for a bird survey that counts. Banding 2010, 42, 32–39. [Google Scholar]
- DeSante, D.F.; Burton, K.M.; Kaschube, D.R.; Velez, P.; Froehlich, D.; Albert, S. MAPS Manual: 2021 Protocol; The Institute for Bird Populations: Petaluma, CA, USA, 2021. [Google Scholar]
- DeSante, D.F.; Kaschube, D.R.; Saracco, J.F. Vital Rates of North American Landbirds. 2015. Available online: https://www.vitalratesofnorthamericanlandbirds.org
- Ellwood, E.R.; Gallinat, A.; Primack, R.B.; Loyd-Evans, T.L. Autumn migration of North American landbirds. In Phenological Synchrony and Bird Migration: Changing Climate and Seasonal Resources in North America. Studies in Avian Biology (No. 47); Wood, E.M., Kellermann, J.L., Eds.; CRC Press: Boca Raton, FL, USA, 2015; pp. 193–205. [Google Scholar]
- McWilliams, S.R.; Guglielmo, C.; Pierce, B.; Klaassen, M. Flying, fasting, and feeding in birds during migration: A nutritional and physiological ecology perspective. J. Avian Biol. 2004, 35, 377–393. [Google Scholar] [CrossRef][Green Version]
- Klaassen, R.H.G.; Hake, M.; Strandberg, R.; Koks, B.J.; Trierweiler, C.; Exo, K.; Bairlein, F.; Alerstam, T. When and where does mortality occur in migratory birds? Direct evidence from long- term satellite tracking of raptors. J. Anim. Ecol. 2014, 83, 176–184. [Google Scholar] [CrossRef] [PubMed]
- Sergio, F.; Tavecchia, G.; Tanferna, A.; Blas, J.; Blanco, G.; Hiraldo, F. When and where mortality occurs throughout the annual cycle changes with age in a migratory bird: Individual versus population implications. Sci. Rep. 2019, 9, 17352. [Google Scholar] [CrossRef] [PubMed][Green Version]
- Deppe, J.L.; Ward, M.P.; Bolus, R.T.; Diehl, R.H.; Celis-Murillo, A.; Zenzal, T.J., Jr.; Moore, F.R.; Benson, T.J.; Smolinsky, J.A.; Schofield, L.N.; et al. Fat, weather, and date affect migratory songbirds’ departure decisions, routes, and time it takes to cross the Gulf of Mexico. Proc. Natl. Acad. Sci. USA 2015, 112, E6331–E6338. [Google Scholar] [CrossRef] [PubMed][Green Version]
- Sjöberga, S.; Alerstama, T.; Åkessona, S.; Schulz, A.; Weidauer, A.; Coppack, T.; Muheima, R. Weather and fuel reserves determine departure and flight decisions in passerines migrating across the Baltic Sea. Anim. Behav. 2015, 104, 59–68. [Google Scholar] [CrossRef]
- Pilastro, A.; Magnani, A. Weather conditions and fat accumulation dynamics in pre-migratory roosting Barn Swallows Hirundo rustica. J. Avian Biol. 1997, 28, 338–344. [Google Scholar] [CrossRef]
- Cornelius, J.M.; Hahn, T.P.; Robart, A.R.; Vernasco, B.J.; Zahor, D.L.; Glynn, K.J.; Navis, C.J.; Watts, H.E. Seasonal patterns of fat deposits in relation to migratory strategy in facultative migrants. Front. Ecol. Evol. 2021, 9, 691808. [Google Scholar] [CrossRef]
- New Mexico Department of Game and Fish. Starvation , Unexpected Weather to Blame in Mass Migratory Songbird Mortality. 2020. Available online: https://content.govdelivery.com/accounts/NMDGF/bulletins/2afbc3e?reqfrom=share
- Johnson, K. The Southwest Is Facing an ‘Unprecedented’ Migratory Bird Die-Off. 2020. Audubon Magazine. Available online: https://www.audubon.org/news/the-southwest-facing- unprecedented-migratory-bird-die.
- Waller, E.K.; Crimmins, T.M.; Walker, J.J.; Posthumus, E.E.; Weltzin, J.F. Differential changes in the onset of spring across US National Wildlife Refuges and North American migratory bird flyways. PLoS ONE 2018, 13, e0202495. [Google Scholar]
- Gustafson, M.E.; Hildenbrand, J.; Metras, L. The North American Bird Banding Manual. Version 1.0. 1997. Available online: https://www.pwrc.usgs.gov/bbl/manual/ .
- Pyle, P. Identification Guide to North American Passerines; Slate Creek Press: Bolinas, CA, USA, 1997; Volume 1. [Google Scholar]
- Liknes, E.T.; Swanson, D.L. Phenotypic flexibility of body composition associated with seasonal acclimatization in passerine birds. J. Therm. Biol. 2011, 36, 363–370. [Google Scholar] [CrossRef]
- R Core Team. R: A Language and Environment for Statistical Computing; R Foundation for Statistical Computing: Vienna, Austria, 2021. [Google Scholar]
- Burnham, K.P.; Anderson, D.R. Model Selection and Multimodel Inference—A Practical Information- Theoretic Approach, 2nd ed.; Springer: New York, NY, USA, 2002. [Google Scholar]
- Belsley, D.A.; Kuh, E.; Welsch, R.E. Regression Diagnostics: Identifying Influential Data and Sources of Collinearity; John Wiley and Sons: New York, NY, USA, 1980. [Google Scholar]
- Birds of North America; Rodewald, P. (Ed.) Cornell Laboratory of Ornithology: Ithaca, NY, USA; Available online: https://birdsna.org .
- The Climate Data Guide: Palmer Drought Severity Index (PDSI); Dai, A., National Center for Atmospheric Research Staff. Available online: https://climatedataguide.ucar.edu/climate- data/palmer-drought-severity-index-pdsi .
- McCullough, J. The Data behind Mysterious Bird Deaths in New Mexico. 2020. American Birding Association, North American Birds Field Ornithology. Available online: https://www.aba.org/the- data-behind-mysterious-bird-deaths-in-new-mexico/ .
Appendix-A Source Code
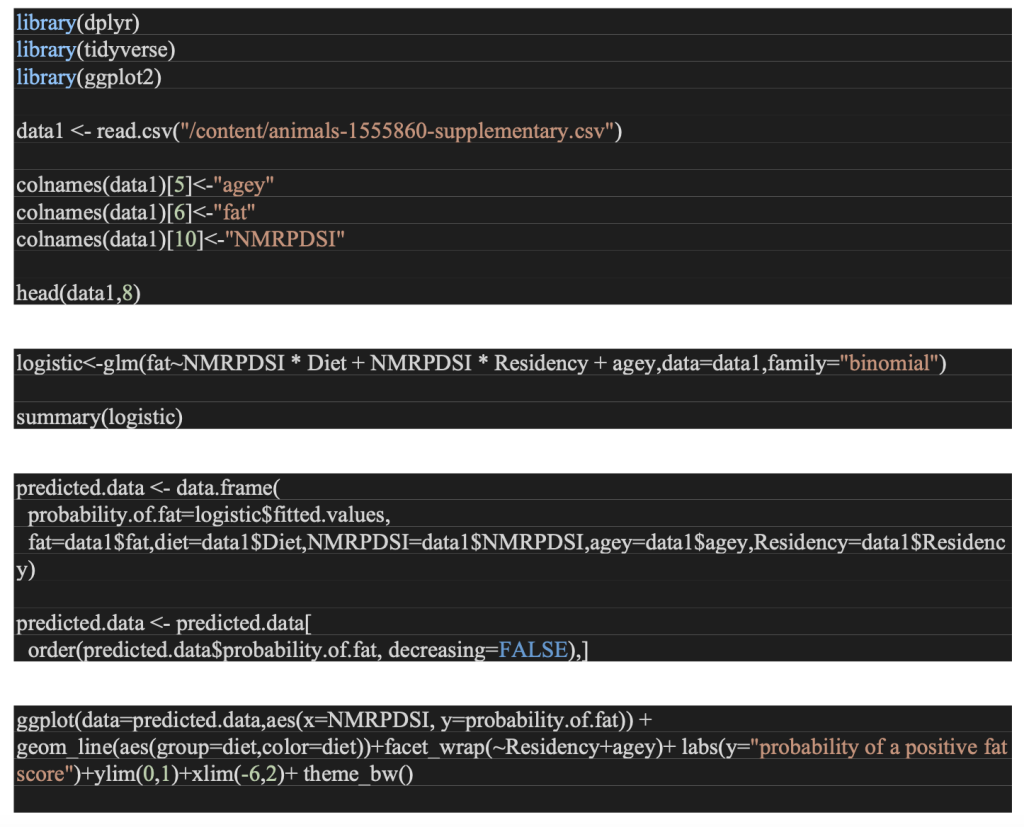
Appendix-B Screenshots
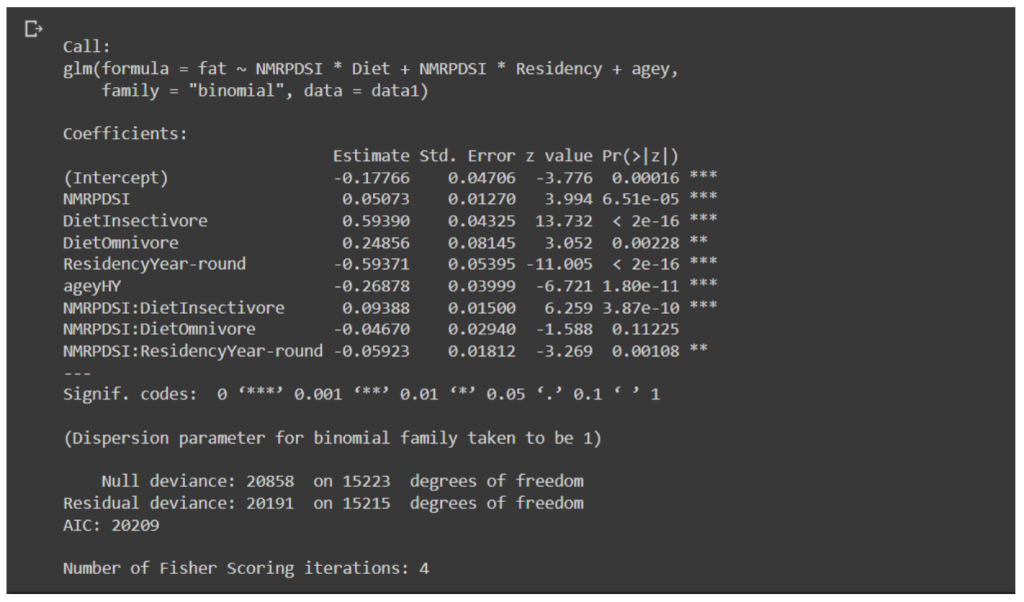
Fig B.1 Summary of logistic model
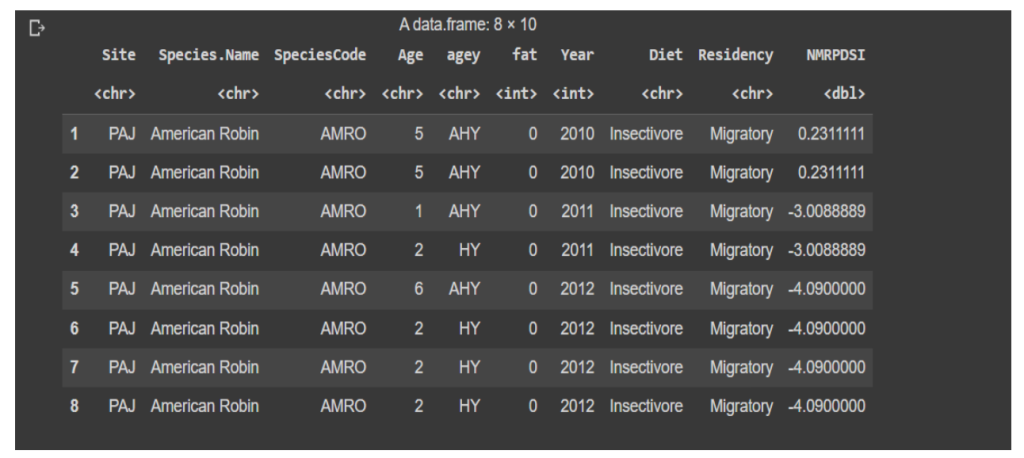
Fig B.2 Dataset for Logistic Regression
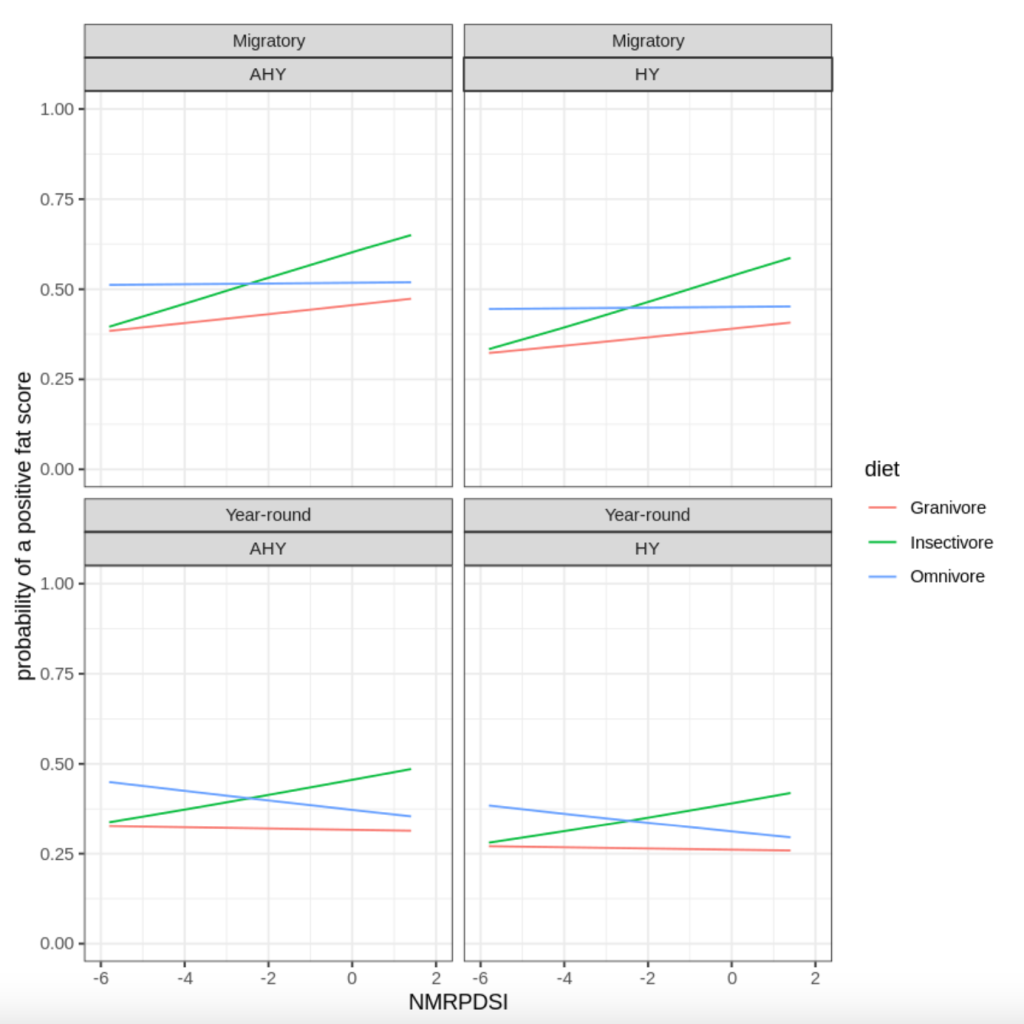
Fig B.3 Results of the model grouped by Residency and Hatch-year
About the author
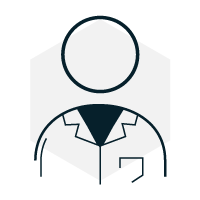
Akshan Krithick
Akshan is currently pursuing undergraduate in Computer Science and Engineering. He has a keen interest on all things Data Science and is motivated to contribute to the field now and in the future.